Rethinking risk ratings: a collaborative data-driven approach
Customer Risk Ratings (CRRs) play a central role in anti-money laundering and counter-terrorist financing (AML/CFT) strategies. But in many institutions, the way CRRs are calculated hasn’t kept up — they are still based on static data, human judgment, and outdated assumptions. The result? Misclassified customers, inefficiencies, and growing regulatory pressure.
In collaboration with Richard Hills from K2 Integrity, we’ve been looking at what a more accurate, transparent, and scalable approach to CRRs could look like — using behavioral data, machine learning, and privacy-preserving collaboration to build more intelligent AML systems.
You can download the guide here now: A Collaborative Approach to Customer Risk Assessment 📕
The takeaway? It’s time to move from isolated decision-making to shared intelligence.
Limitations of traditional risk rating methods
Most institutions still rely on customer risk rating models built around static KYC data, qualitative scoring matrices, and human interpretation. While these methods may be familiar, they create several ongoing problems:
- 🟣Subjectivity: Different analysts often apply different logic or interpretations
- 🟣Limited inputs: Risk scores are based on snapshots of identity, not real behavior
- 🟣Lack of transparency: The logic behind scores is hard to audit or explain
- 🟣Risk of bias: Decisions may be influenced by irrelevant or inconsistent factors
These flaws are well documented. The FCA has highlighted oversimplified CRR models as a weakness in the UK’s AML defenses, while FinCEN’s enforcement actions point to concerns about the quality and consistency of customer risk assessments in the U.S.
Related reading: Banking on collaboration: overcoming the stalemate in AML innovation |
Why CRRs matter more than ever
While CRRs are a compliance requirement, they shape how institutions manage customer risk throughout the entire lifecycle. A poorly rated customer might slip through enhanced due diligence or trigger unnecessary alerts. A well-rated one helps focus resources where they matter most.
When done right, CRRs:
✅Stronger AML & regulatory compliance
✅Identify high risk customers more efficiently and effectively
✅Inform proportional due diligence and monitoring
✅Reduce false positives and compliance overhead
✅Support audit-readiness with traceable logic
But when they’re based on outdated inputs or inconsistent assumptions, the consequences ripple across the compliance function — from inefficient processes to regulatory exposure.
Modernizing CRR through machine learning
Fortunately, there’s a simple solution. Machine learning (ML) provides a more consistent, data-driven way to assess customer risk. Instead of relying on static KYC forms, ML models analyze patterns in behavior – such as transaction activity, location, and network interactions — to identify indicators of elevated risk.
1. Risk scores based on real-world behavior 🗺
ML systems detect subtle changes and anomalies in customer activity over time, which can help identify emerging risks earlier. These models don’t rely on assumptions—they learn from the data.
2. Consistency at scale 📈
Unlike manual scoring, ML applies the same set of criteria to every customer. That means fewer discrepancies between analysts and a more predictable way to assess risk across the portfolio.
3. Reduced bias ⏬️
With the right controls in place, ML models can avoid using inputs that could lead to discriminatory outcomes — focusing instead on factors directly linked to risk. This helps institutions align with both ethical standards and regulatory expectations.
4. Auditability 📄
Modern ML tools allow institutions to document how a model reached a particular outcome, including what data was used, how it was weighted, and why the risk score was assigned. This kind of transparency is essential when explaining decisions to internal stakeholders or regulators.
“Machine learning gives us a way to apply consistent, data-driven criteria across all customer profiles — and to show exactly how we got there,” says Ajit Tharaken. “That level of clarity is something both compliance teams and regulators have been asking for.”
Related reading: How AI helps governments and businesses tackle AML |
Collaborating without sharing data: How federated learning works
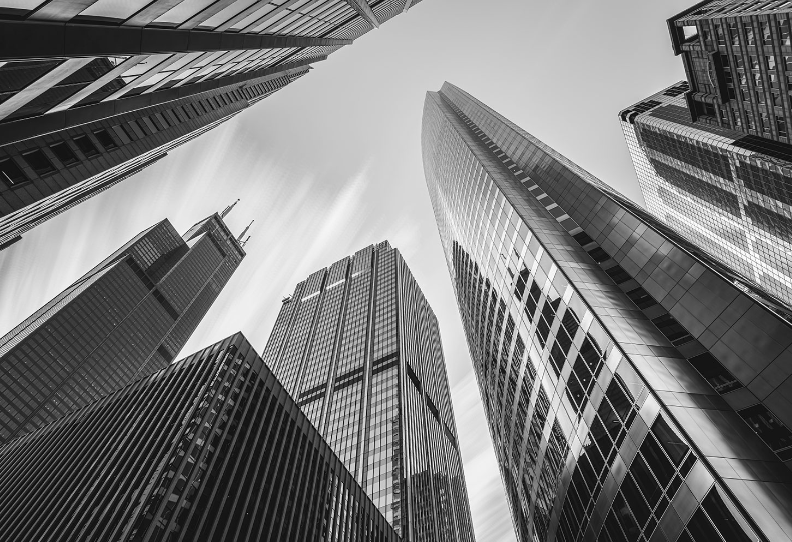
One of the biggest challenges in improving CRR is data fragmentation. Each institution only sees part of the picture — and data sharing between organizations is often restricted by privacy laws or internal policy.
Federated Learning (FL) offers a way around this. It allows financial institutions to train models collaboratively, without exposing any sensitive data. Each institution keeps its data locally and shares only encrypted model updates — not raw data — with a central system that aggregates the results.
This approach means institutions can:
➡Benefit from a broader range of data patterns
➡Improve model performance with no compromise on privacy
➡Build a shared understanding of financial crime risk
Use cases for federated learning include shared CRR model development, cross-institution screening for high-risk entities, and reducing false positives in transaction monitoring — all without needing to move or pool data centrally.
“Federated Machine Learning (FML) offers a secure and scalable way for financial institutions to collaborate without sharing sensitive customer data,” explains Ajit Tharaken. “By exchanging only model updates—not raw data—it enables the creation of a collective, high-performing model that preserves privacy and enhances compliance.”
Related reading: How AI federated machine learning strengthens AML defenses |
Aligning with regulatory expectations
Of course, regulators are paying close attention to how financial institutions use automation and AI in risk decisions. The direction of travel is clear: models must be explainable, well-governed, and demonstrably fair.
ML based CRRs – especially those developed through federated learning – can help institutions meet those expectations by offering:
🟣Clear audit trails: Showing how risk scores were generated and which inputs were used
🟣Governance frameworks: Including version control, documentation, and testing protocols
🟣Fairness safeguards: Ensuring models exclude irrelevant or biased inputs
🟣Transparency: So institutions can explain decisions confidently during audits or supervisory reviews
Institutions that adopt this approach are better placed to respond to regulatory scrutiny — and to evolve their compliance programs as expectations continue to change.
A smarter, more connected future for AML
Improving CRR means more than better algorithms. Organizations need to build a more collaborative, evidence-based approach to managing risk.
Federated learning makes this possible. It allows financial institutions to benefit from shared insights without exposing sensitive data. That changes what’s possible. Institutions can learn from each other, adapt faster to new threats, and reduce duplicated effort across the industry.
Plus, as adoption of ML and FL increases, there’s potential to:
🟣Standardize CRR models across institutions
🟣Improve risk signal quality for law enforcement and regulators
🟣Build a more adaptive, joined-up AML ecosystem
This is how the industry moves from fragmented controls to shared resilience.
Final thoughts
Traditional CRRs are no longer fit for purpose. They rely too heavily on static information, inconsistent judgment, and opaque logic — all of which create compliance challenges and limit effectiveness.
Machine learning offers a more consistent auditable way to assess customer risk based on how people behave, not just what’s on file. Federated learning takes it further, creating opportunities for collaboration without sacrificing privacy or control.
Together, these approaches make it possible to build smarter, more aligned, and more proactive compliance frameworks, helping financial institutions manage risk more effectively and keep pace with regulators’ expectations.
Want to rethink CRR in your institution? Consilient works with financial institutions across the U.S. to deploy federated learning models that improve AML detection, reduce false positives, and support smarter compliance decisions. If you’re considering how to modernize CRR — or any other part of your AML framework — we’d love to hear from you.
📚 Further reading
Beyond the 1%: How AI Federated Learning is catching more financial criminals
Building trust in finance: How federated learning strengthens AML defenses
How model intelligence is transforming financial crime detection