Our Federated solution
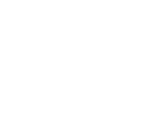
Play
Correspondent Banking model
Federated learning model for enhanced risk management in
Correspondent Banking
Introducing our cutting-edge federated learning model, specifically designed to address the unique risks associated with correspondent banking customers.
This model significantly advances transaction monitoring, customer and enhanced due diligience activities by identifying underlying customer risks. Built in collaboration with international banking institutions and leveraging high risk behaviors and patterns along with Suspicious Activity Reports (SARs).
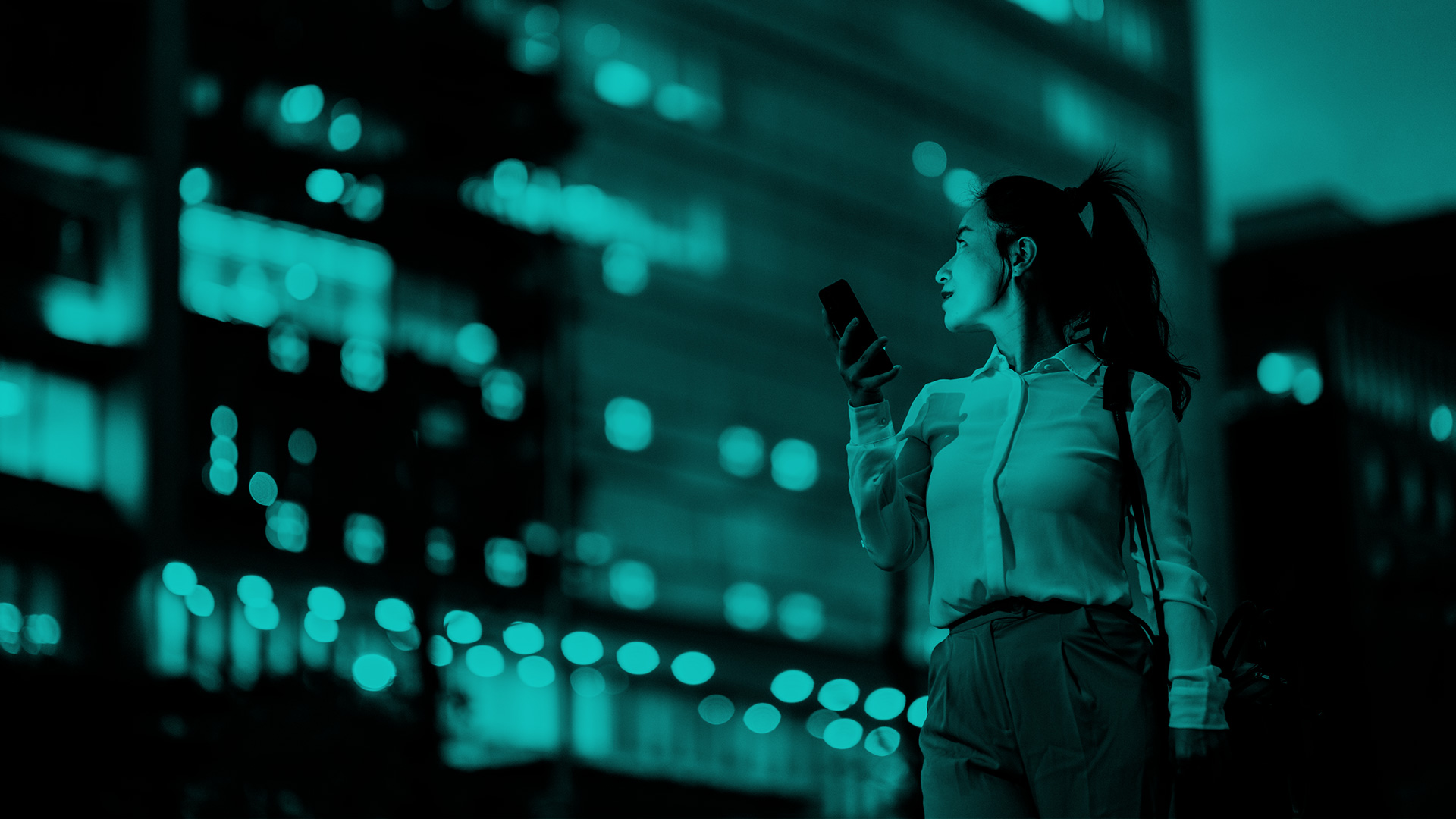
Key features
Consilient allows clients to understand the behaviors of their Correspondent Bank's customers.
Consilient utilizes 12 months of behaviors to offer a level of risk for each of the Correspondent Bank's customers, along with key red flags such as identification nesting and high-risk jurisdiction activities.
This model benefits from understanding the behaviors of high risk customers of a bank's Correspondent Bank's customers.
By aggregates knowledge federated learning enhances the understanding of high risk wires and transactions without requiring direct data sharing.
This collaborative approach enhances the model’s ability to identify patterns and risks that might be missed by systems relying on isolated datasets.
Continuous learning is crucial for machine learning models' ongoing relevance and effectiveness, particularly in dynamic and evolving environments. It allows them to adapt to new patterns and trends.
Adversarial evolution: In areas like AML, criminals actively adapt their methods to evade detection. Continuous learning ensures machine learning models stay ahead of these tactics. Regular updates refine the model's understanding, improving accuracy and reducing errors in prediction or classification.
In areas like AML, criminals actively adapt their methods to evade detection. Continuous learning ensures machine learning models stay ahead of these tactics.
Regular updates refine the model's understanding, improving accuracy and reducing errors in prediction or classification.
Federated learning allows AI models to be trained directly on local datasets within the confines of an organization's secure infrastructure. Only the aggregated model updates, not raw data, are shared across participants.
By avoiding centralized data pooling, federated learning minimizes the risk of data breaches, reducing the security concerns often accompanying inter-agency or inter-institutional collaborations.
Consilient uses the Ensembling technique to address key challenges in developing precise and efficient models. Ensembling in federated learning leverages the strengths of local models while mitigating their weaknesses.
By integrating diverse insights without compromising privacy or efficiency, ensembling creates a robust, accurate, and scalable approach to federated learning,
Traditional AML machine learning models often rely on SAR training data. However, many SARs are filed defensively rather than in response to genuine risk.
The Consilient approach instead incorporates multiple typology models, many trained on specific high-risk behaviors like those of MSBs or precious metals dealers. This makes it easier to screen for a broader range of risks.
Key benefits
Dramatic reduction in false positives
Traditional transaction monitoring systems often flood compliance
teams with false positive alerts, leading to inefficiencies and increased
operational costs.
Our Correspondent Bank model has demonstrated over a 76%
reduction in false positive alerts from beneficiary and counter-parties
allowing your team to focus on genuine threats and streamline the
review process.
Improved detection capabilities
The model’s sophisticated algorithms have shown a remarkable 244% increase in detecting suspicious activities, ensuring a more comprehensive and accurate identification of potential risks.
This improvement significantly enhances your bank's ability to prevent financial crimes and maintain regulatory compliance.
Dramatically reduce operational costs
The federated learning model was estimated to reduce annual review costs by 92% in a recent pilot program.
By minimizing false positives and improving detection accuracy, your bank can allocate resources more efficiently and reduce the overall cost of compliance operations.
Dramatic reduction in false positives
Traditional transaction monitoring systems often flood compliance
teams with false positive alerts, leading to inefficiencies and increased
operational costs.
Our Correspondent Bank model has demonstrated over a 76%
reduction in false positive alerts from beneficiary and counter-parties
allowing your team to focus on genuine threats and streamline the
review process.
Improved detection capabilities
The model’s sophisticated algorithms have shown a remarkable 244% increase in detecting suspicious activities, ensuring a more comprehensive and accurate identification of potential risks.
This improvement significantly enhances your bank's ability to prevent financial crimes and maintain regulatory compliance.
Dramatically reduce operational costs
The federated learning model was estimated to reduce annual review costs by 92% in a recent pilot program.
By minimizing false positives and improving detection accuracy, your bank can allocate resources more efficiently and reduce the overall cost of compliance operations.
Pin-point risks in your Correspondent Bank channel
Our Federated Learning model represents the future of transaction monitoring and risk management for correspondent banking.
Where any single bank has limited data to build robust models, federated learning changes that paradigm by leveraging collaboration to create the most extensive data set available for model development.
Correspondent Banking from Consilient significantly reduces false positives, improves detection rates, and cuts costs. It enables your bank to stay ahead of evolving financial crimes while maintaining compliance and operational efficiency.