Consilient transforms risk
management.
Between $800 billion and $2 trillion in illicit transactions is laundered through the financial system each year, with less than 1% of the transactions detected.
Our solution is 3x more efficient and detects 10% more cases than the same company only model.
The Consilient Solution
Consilient delivers the first Federated Learning solution for anti-money laundering and financial crime prevention.
It allows institutions, regulators and jurisdictions to share behavioral insights to improve risk management without the challenges of sharing data.
For more about Consilient’s Federated Learning solution, watch our video here.
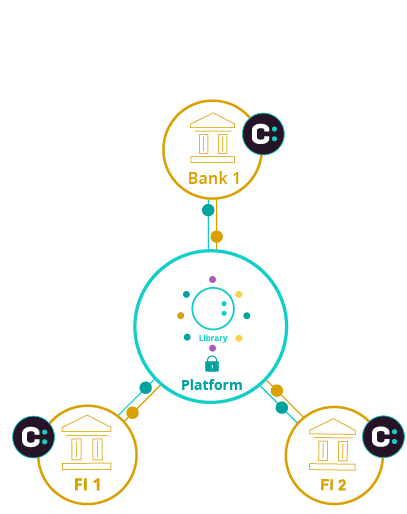
Federated Learning
Our approach
Federated Learning optimizes models by sharing industry insights. Our approach allows institutions to leverage the most up-to-date and optimal models for anti-money laundering and specific financial crime risks.
Institutions bring those models in from our secure platform, deploying locally, in accordance with all model risk management approaches.
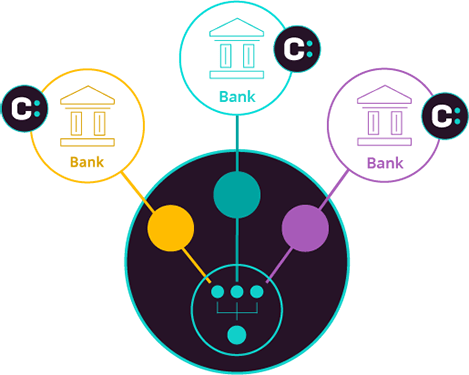
Our models
Our models identify high risk entity behaviors, allowing an organization to identify accounts that are behaving anomalously and in a way that appears to be associated with specific high-risk categories.
The models are trained, at each organization, using a privacy protected approach and travels back to the Consilient platform, where it is aggregated, validated and orchestrated with other versions, creating a new “champion” model, which is ready to be federated for even more performance benefit.
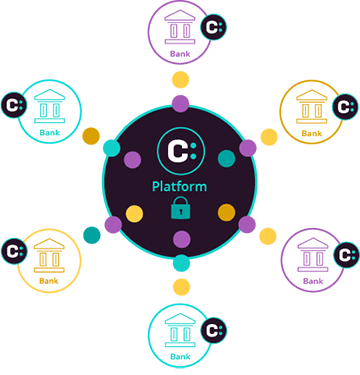
Bringing insights together
Consilient brings together insights from the community of financial institutions allowing organizations to benefit from the industry view of anti-money laundering and financial crime intelligence.
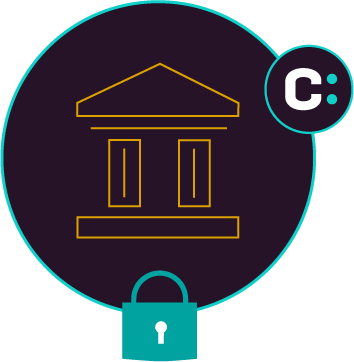
Privacy-preserving technologies
Ensuring data privacy is at the heart of our solution.
Privacy-preserving technologies are deployed to allow our models to continuously improve performance safely and securely, which is then deployable to all participants in the Consilient network.
Our approach
Federated Learning optimizes models by sharing industry insights. Our approach allows institutions to leverage the most up-to-date and optimal models for anti-money laundering and specific financial crime risks.
Institutions bring those models in from our secure platform, deploying locally, in accordance with all model risk management approaches.
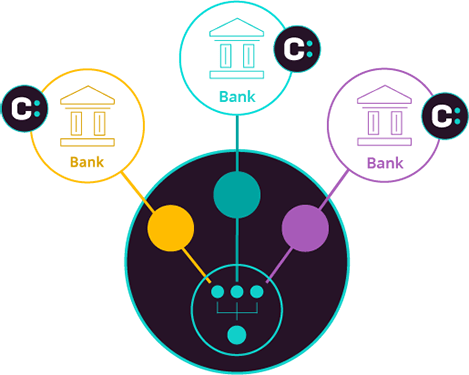
Our models
Our models identify high risk entity behaviors, allowing an organization to identify accounts that are behaving anomalously and in a way that appears to be associated with specific high-risk categories.
The models are trained, at each organization, using a privacy protected approach and travels back to the Consilient platform, where it is aggregated, validated and orchestrated with other versions, creating a new “champion” model, which is ready to be federated for even more performance benefit.
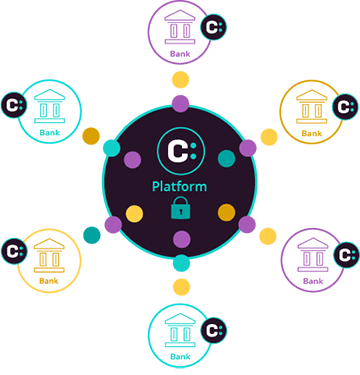
Bringing insights together
Consilient brings together insights from the community of financial institutions allowing organizations to benefit from the industry view of anti-money laundering and financial crime intelligence.
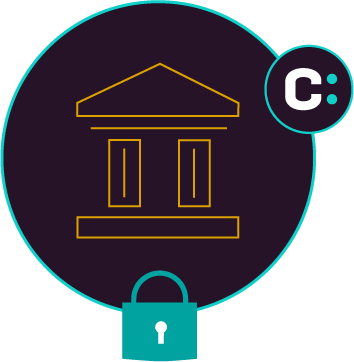
Privacy-preserving technologies
Ensuring data privacy is at the heart of our solution.
Privacy-preserving technologies are deployed to allow our models to continuously improve performance safely and securely, which is then deployable to all participants in the Consilient network.
What market and organizational challenges can Consilient overcome?
The Consilient solution has been designed to combat these challenges facing firms and regulators in an increasingly complex financial crime threat landscape:
Risk management
Navigating the risk landscape can be a challenging and costly procedure for many institutions.
Effectively detecting, investigating and reporting suspicious activity requires significant resources, complex processes and high management overhead.
Privacy vs effectiveness
Current industry tools are limited in their ability to learn across institutions. Information sharing is mostly anecdotal and limited to protect data privacy.
Data protection risks and complex systems means that insights cannot be shared across institutions. Limiting the benefits of knowledge sharing.
Existing detection processes
Criminal enterprises are continually advancing and practiced in their evasion of financial crime detection.
Long-standing industry standard detection systems lack sophistication in their rule based detection of suspicious behavior.
Limited risk coverage
Limited internal data can lead to the inability to build specific model types, and poorly performing models which in turn inhibits detection rates.
Bringing effectiveness and risk discovery challenges.
Efficiency
Current systems, processes and models carry extraordinary management of time, costs and resources.
Yet still leads to high false positive rates and case volumes.
Risk management
Navigating the risk landscape can be a challenging and costly procedure for many institutions.
Effectively detecting, investigating and reporting suspicious activity requires significant resources, complex processes and high management overhead.
Privacy vs effectiveness
Current industry tools are limited in their ability to learn across institutions. Information sharing is mostly anecdotal and limited to protect data privacy.
Data protection risks and complex systems means that insights cannot be shared across institutions. Limiting the benefits of knowledge sharing.
Existing detection processes
Criminal enterprises are continually advancing and practiced in their evasion of financial crime detection.
Long-standing industry standard detection systems lack sophistication in their rule based detection of suspicious behavior.
Limited risk coverage
Limited internal data can lead to the inability to build specific model types, and poorly performing models which in turn inhibits detection rates.
Bringing effectiveness and risk discovery challenges.
Efficiency
Current systems, processes and models carry extraordinary management of time, costs and resources.
Yet still leads to high false positive rates and case volumes.
Use cases
Enhanced control
Consilient’s High-Risk Entity model can be deployed as the foundational AML and countering the financing of terrorism (CFT) model for customer risk management, identifying potential new high-risk entities and anomalous behaviors of existing entities.
Our solution works on a systematic basis to enabling active risk management.
Existing tool augmentation
Financial institutions can utilize Consilient models to augment existing tools and processes, enhancing the identification of specific types of threats and risks. Augmentation enables detection and coverage of previously hard to identify and regulatory priority risk types.
Augmentation creates a real benefit in the identification of new high-risk entities not found by existing tools.
Assurance
Financial organizations can use our models as a second line of defense to validate existing risk and threat identification.
Banking functions such as audit and risk can use Consilient as an empirical view of risk to assure themselves existing approaches are functioning.
Standardized management
Organizations can be siloed through acquisition, brand and new divisional developments. In these scenarios money laundering risk controls may differ.
Utilizing Federated Learning can ensure that the discovery of risk is standardized across the organization. Allowing the risk function clear oversight of all geographies and providing regulators with demonstrable compliance across the business.
Enhanced control
Consilient’s High-Risk Entity model can be deployed as the foundational AML and countering the financing of terrorism (CFT) model for customer risk management, identifying potential new high-risk entities and anomalous behaviors of existing entities.
Our solution works on a systematic basis to enabling active risk management.
Existing tool augmentation
Financial institutions can utilize Consilient models to augment existing tools and processes, enhancing the identification of specific types of threats and risks. Augmentation enables detection and coverage of previously hard to identify and regulatory priority risk types.
Augmentation creates a real benefit in the identification of new high-risk entities not found by existing tools.
Assurance
Financial organizations can use our models as a second line of defense to validate existing risk and threat identification.
Banking functions such as audit and risk can use Consilient as an empirical view of risk to assure themselves existing approaches are functioning.
Standardized management
Organizations can be siloed through acquisition, brand and new divisional developments. In these scenarios money laundering risk controls may differ.
Utilizing Federated Learning can ensure that the discovery of risk is standardized across the organization. Allowing the risk function clear oversight of all geographies and providing regulators with demonstrable compliance across the business.
What customer benefits does Consilient deliver?
Federated Learning is proven to enable clients to reduce effort by two thirds with no decrease in effectiveness.
Incorporation of market-wide learning and a diverse set of models improves identification of risks and suspicious activity filing.
Utilizing Federated Learning can significantly reduce cost of modeling, processes, control systems, testing and operations.
Continuous review is possible due to greater accuracy. Identification of high-risk customers quickly and systematically.
Identify customers with anomalous behavior that present differently to type and whose behavior is high risk.
Improved identification of suspicious activity. Demonstrable best in class risk management and regulatory compliance.