Beyond the 1%: How AI Federated Learning is catching more financial criminals
Less than 1% of actual illicit transactions are detected globally. This is a hard fact to ignore. It leaves firms vulnerable to increasingly sophisticated criminal networks, despite enormous resources poured into anti-money laundering (AML) efforts.
Why is this happening? While criminal tactics evolve rapidly, the tools used by financial institutions haven’t kept pace. Traditional AML systems, often reliant on siloed data and manual processes, are both resource-intensive and prone to error. Criminals exploit these gaps, allowing illicit funds to flow freely across borders. Are senior managers stuck in their ways?
But what if there was a way to change all this?
Enter AI Federated Learning—the leading edge solution for tackling financial crime. Unlike traditional ML models, AI Federated Learning enables secure collaboration between institutions, allowing them to share behavioral insights and detect broader patterns of suspicious activity –without compromising data privacy.
In this post, we‘ll cover how AI Federated Learning can raise detection rates and close the gaps in financial crime prevention.
Why traditional AML systems are losing the fight
Current AML systems are falling short. Despite significant investments, firms are struggling to keep pace with financial criminals. Here’s why:
🚩Manual processes and human error
Many financial institutions still rely heavily on manual processes for AML tasks. Teams of skilled analysts review flagged transactions and prepare Suspicious Activity Reports (SARs). While thorough, this approach is time-consuming and costly. Some banks employ thousands of analysts, driving up operational expenses. In the U.S. alone, financial institutions spend approximately $180 million annually on analyst salaries for AML tasks—a substantial investment that, unfortunately, hasn’t yielded proportional improvements in detection rates.
🚩Siloed data and traditional Machine Learning
While some institutions have adopted machine learning models to enhance efficiency, these systems often operate in isolation. Traditional ML models are restricted. They can only analyze data from a single institution, missing broader patterns of financial crime that span multiple organizations. And this limits the models’ effectiveness, particularly against sophisticated criminals who operate across borders and institutions.
🚩Overwhelming false positives
Even with newer technology, false positives remain a significant challenge. Current AML systems generate a high volume of alerts, many of which turn out to be benign. Compliance teams must carefully sift through these alerts to identify genuine threats, a process that delays investigations and stretches resources. The sheer volume of false alerts can inadvertently create blind spots, as institutions focus on managing the high alert volume rather than detecting actual criminal activity.
Firms need a better approach to AML—one that can enhance detection capabilities for all financial service providers.
AI Federated Learning: The modern approach to AML
AI Federated Learning is a major leap forward in AML. It has proven to reduce false positives by over 80% and increase detection rates by up to 300%. By tapping into insights across institutions, AI Federated Learning allows for more accurate threat identification and faster response times.
Key features of AI Federated Learning:
- 🟣Enables model training across multiple organizations without sharing data
- 🟣Preserves data privacy while allowing institutions to benefit from collective insights
- 🟣Improves detection of complex, multi-jurisdictional financial crimes
Applications in financial crime detection
Here’s where AI Federated Learning shines:
#1. Cross-border money laundering schemes
AI Federated Learning enables the detection of sophisticated laundering operations that exploit gaps between different jurisdictions. By analyzing patterns across multiple institutions, it can identify coordinated activities that might otherwise go unnoticed.
#2. High-risk entity detection
This approach improves the identification of high-risk customers or businesses by pooling insights from multiple sources. Behavior that might not appear suspicious within a single institution can be recognized as high-risk when viewed in a broader context.
#3. Uncovering hidden anomalies
AI Federated Learning provides a wider perspective for detecting unusual activities. Transactions or behaviors that seem normal in isolation may reveal suspicious patterns when analyzed across multiple institutions, enhancing the detection of complex financial crimes.
By leveraging AI Federated Learning, financial institutions can significantly improve their AML capabilities. This collaborative approach, while preserving individual data privacy, creates a more robust defense against increasingly sophisticated financial crimes.
The global impact of improved detection
Raising the detection rate of illicit transactions from the current 1% to significantly higher levels would have profound effects on the global financial system. This improvement enhances government agencies’ ability to catch criminals and can reshape how financial institutions and regulators approach the fight against financial crime–across the globe. Here’s how:
▶Deterring crime
Improved detection rates could make the financial system significantly less appealing for criminal activities. As the risk of being caught increases, criminal networks may be forced to reconsider their strategies. A more robust financial crime detection system, powered by AI Federated Learning, could serve as a powerful deterrent, potentially reducing the volume of illicit transactions across the globe.
▶Strengthening regulatory compliance
Enhanced detection capabilities could lead to markedly better compliance with regulatory standards. Financial institutions often face penalties for failing to detect or report suspicious activity. More accurate and efficient identification processes could not only reduce these penalties but also demonstrate to regulators that institutions are leveraging cutting-edge AML tools to meet and exceed compliance requirements.
▶Systemic efficiency gains
AI Federated Learning doesn’t just improve detection—it can also streamline operations. By reducing false positives and automating aspects of the AML process, institutions can allocate their human expertise more effectively, focusing on genuine threats. This shift toward more automated, accurate systems can create lasting efficiency gains, potentially reducing overall compliance costs while improving effectiveness.
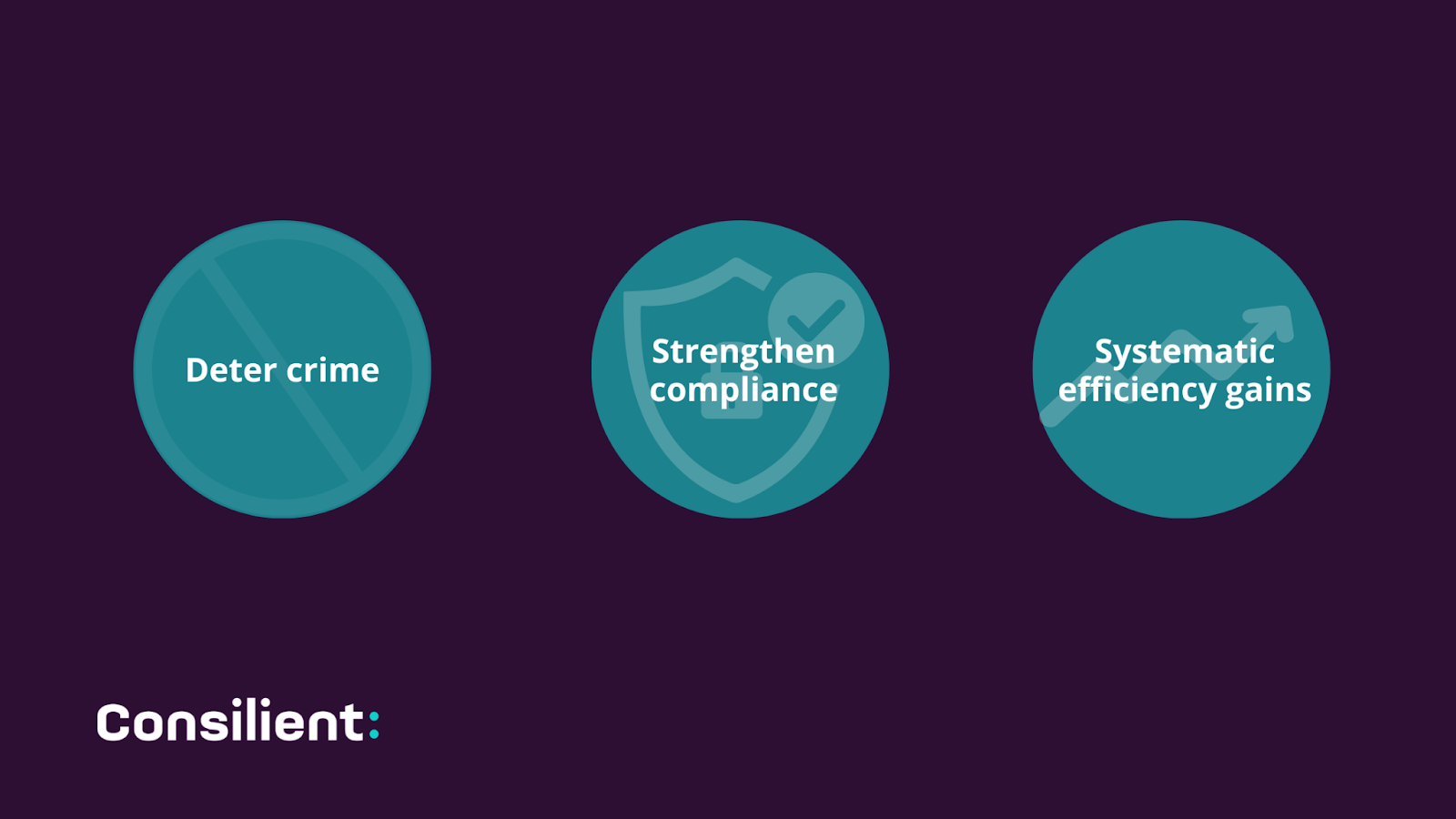
The future of financial crime detection
As we look to the future of financial crime detection, one thing is clear: the status quo is no longer sufficient. Financial criminals are becoming increasingly sophisticated. In response, our detection and prevention tools must evolve at an equal pace.
AI Federated Learning offers financial institutions the power to detect fraudulent activities, address longstanding vulnerabilities, and close the gaps that criminals have long exploited. With this capability, firms are better equipped to protect their assets, safeguard their customers, and contribute to a more secure global financial system.
It’s time for firms to change. By adopting AI Federated Learning, they can strengthen their defenses against increasingly complex criminal networks, reduce regulatory exposure, and improve operational efficiency. Talk to us if you are keen to learn more about AI Federated Learning. We’d genuinely love to help.