How AI Federated Machine Learning strengthens AML defenses
Financial institutions invest billions in anti-money laundering (AML) programs, yet they face two critical challenges that undermine their effectiveness. First, fragmented approaches across institutions create exploitable gaps in the financial system through new and undiscovered changes in suspicious behaviors. Second, the lack of feedback on Suspicious Activity Reports (SARs) leaves banks operating in the dark and potentially raising SARs to demonstrate they have robust and appropriate controls for regulators.
This post examines how federated learning (FL)—a new technology that extends the capabilities of machine learning—can bridge this long-standing gap in AML effectiveness.
Let’s get straight into it.
The cost of fragmentation in AML
Between $800 billion and $2 trillion in illicit transactions flow through the financial system annually. Despite enormous investments in detection systems, less than 1% of these transactions are identified as suspicious.
False positive rates for AML alerts reach as high as 98%. No other industry could survive while discarding 98% of its output—yet this is the reality for financial crime detection.
The challenge starts with fragmentation. Financial institutions develop and implement AML controls independently, creating significant inconsistencies:
❌ Different transaction monitoring systems and rules
❌ Varied risk assessment methodologies
❌ Inconsistent alert investigation processes
❌ Disparate data analysis capabilities
The fragmentation problem stems from two systemic issues. First, institutions have to prioritize meeting regulatory requirements over measuring actual effectiveness. Second, and extraordinarily, there’s no unified approach to identifying criminal activity. Which has proven in the past to allow calamitous events to occur and provide criminals with holes in the system to exploit.
While regulators establish the framework, feedback on SAR submissions is rarely provided. This leaves a blind spot where institutions design their AML controls independently, without clear insight into their effectiveness. Ironically, while firms aim to prove that their controls are sound, they have no real way of validating their ultimate effectiveness. Criminal networks then actively exploit these gaps, using institutions with weaker controls as entry points into the broader financial system.
For banks, the impact goes beyond regulatory penalties and reputational damage. When TD Bank’s controls were improperly configured and implemented, it resulted in a record $3 billion fine from U.S. regulators, and the announcement triggered a market value drop of over CAD $11 billion. This sharp decline highlights the financial and reputational consequences that regulatory penalties can have on major institutions. The penalty, related to deficiencies in anti-money laundering practices, is the largest fine ever imposed by U.S. regulators. TD Bank’s case was of course extreme and was an accumulation of a number of issues.
However, market fragmentation and the existing silo approach undermines the effectiveness of even the most sophisticated AML programs.
The SAR black hole: Operating without feedback
Every AML professional knows this scenario: Your team meticulously investigates suspicious activity, files a detailed SAR, and then… nothing. The report disappears into what many call the “SAR black hole,” leaving crucial questions unanswered:
🟣Did this SAR contribute to identifying criminal activity?
🟣Are our detection scenarios properly calibrated?
🟣Should we adjust our investigation procedures?
🟣What emerging threats are we potentially missing?
This feedback void creates a significant challenge. Without knowing which SARs lead to successful investigations, financial institutions can’t effectively:
🟣Refine their detection models
🟣Optimize investigative resources
🟣Adapt to new criminal methodologies
🟣Validate their risk assessment approaches
The impact extends beyond individual institutions. When banks can’t learn from their SAR submissions, the entire industry’s ability to combat financial crime stagnates. Meanwhile, criminal networks continue to evolve their tactics, exploiting this lack of shared intelligence.
Federated Learning: Bridging both gaps
The real-life data speaks for itself: Federated Learning has demonstrably reduced false positives by over 80% and increased detection rates by up to 300%. With U.S. institutions spending $80 billion annually on AML compliance, these efficiency gains represent significant operational value.
But how does Federated Learning achieve these results?
Think of Federated Learning as a secure bridge between institutions. Each bank retains full control over its sensitive data while simultaneously contributing to and gaining insights from a shared intelligence network.
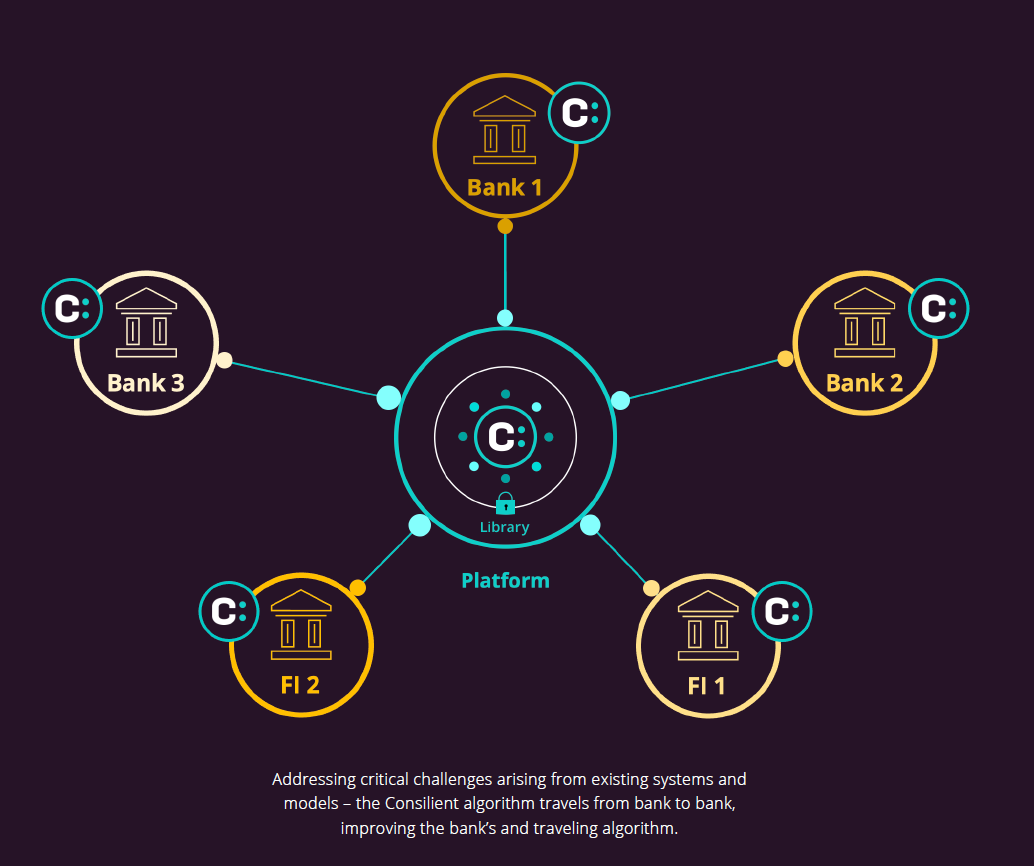
This collaborative approach eliminates the traditional trade-off between data privacy and enhanced detection capabilities. Federated Learning changes this paradigm:
Enhances detection without compromising privacy
⏺Banks learn from peer institutions’ identified financial crime behavioral patterns;
⏺Customer data remains securely within each institution;
⏺Machine learning models improve through learning against the largest data of suspicious activity;
⏺Privacy-preserving architecture ensures data safety and security.
Strengthens detection and reduces costs
⏺Dramatic reduction in false positives;
⏺Lower investigation costs;
⏺Improved investigator efforts and SAR submission quality;
⏺More efficient resource allocation.
Builds industry-wide defense
⏺Unified defense against emerging threats;
⏺Rapid adaptation to new criminal methodologies;
⏺Reduced exploitation of gaps between institutions;
⏺Consistent approach across the financial sector.
Federated Learning tackles one of the financial sector’s most enduring challenges—fragmented AML strategies—by training a machine learning model and aggregating insights across multiple institutions. It enables banks to validate detection methods against industry patterns while maintaining their operational independence. This means institutions can quickly identify emerging threats and adapt strategies based on collective experience, not just their own limited view of suspicious activity.
The result? A financial system where individual institutions maintain their independence while contributing to a collaborative defense against financial crime. Rather than operating in isolation, banks can now validate their approaches against industry-wide patterns and adapt their strategies based on collective insights.
Broader implications for trust: Beyond individual bank security
Federated Learning’s impact extends far beyond individual institution performance metrics.
When banks share intelligence through privacy-preserving collaboration, it fundamentally changes how the financial sector fights crime.
Here’s how it impacts others:
Stakeholder | Key benefits | Impact |
Regulators | ⏺Clear visibility into AML effectiveness across institutions ⏺Data-driven evidence of compliance efforts ⏺Consistent approach to measuring and monitoring outcomes | Enables more effective oversight and reduces systemic risk from disparate systems |
Financial institutions | ⏺Lower risk of regulatory penalties ⏺Reduced compliance and operational costs ⏺Stronger defense against emerging threats | Demonstrates clear ROI and program effectiveness while strengthening AML operations |
Financial system | ⏺Improved detection of cross-border criminal activity ⏺Faster adaptation to new money laundering methods ⏺Reduced exploitation of gaps between institutions | Creates a more resilient system through coordinated defense against financial crime |
Jurisdictional financial investigation units | ⏺Better and more productive SARs ⏺Reduced waste from case overload ⏺SARs that are targeted for the FIU | Enables financial crime investigators to investigate more cases that are meaningful. |
By replacing isolated defenses with coordinated intelligence sharing, financial institutions can now build collective strength against criminal activity.
The future of AML with AI Federated Learning
While criminals collaborate to exploit systemic weaknesses, institutions often fight financial crime in isolation. This has to change. The rates of transaction detection, combined with the high costs emphasize the huge gap that criminals can exploit.
Federated Learning changes all this.
By enabling secure intelligence sharing between institutions, it delivers a significant, measurable impact. With false positives dramatically reduced and suspicious activity detection significantly improved, institutions can now demonstrate enhanced regulatory compliance while strengthening their (cross-border) threat detection capabilities.
As financial crime grows more sophisticated, the choice becomes clear: continue with isolated, silo-based defenses or embrace collaborative intelligence sharing that protects privacy while strengthening industry-wide security.
Talk to us if you are keen to learn more about AI Federated Learning. We’d genuinely love to help.
FAQs
In case you still have questions, we’ll end by covering some Frequently Asked Questions.
How can trust be built if all banks’ AML safeguards are different?
AI Federated learning enables institutions to benchmark their detection patterns against industry-wide data while maintaining data privacy. This allows banks to validate their controls, identify potential gaps, and align with industry best practices through secure collaboration.
Can AML programs improve without direct SAR feedback?
Yes. While direct SAR feedback remains limited, federated learning provides institutions with aggregate insights from across the financial sector. This broader perspective helps banks calibrate their detection systems, refine their investigation processes, and identify emerging criminal patterns more effectively.
How can trust be built when there is no sharing of insights between institutions?
AI Federated Learning technology enables banks to contribute to and learn from collective intelligence without exposing sensitive data. Models learn from patterns across institutions while raw data remains secure within each bank’s environment. This creates a framework for collaboration that meets both privacy and regulatory requirements.