Anti-money laundering (AML) risk management reimagined: The Consilient approach
AML approaches rely heavily on manual processes, just to prepare Suspicious Activity Reports is estimated to cost $180 million annually in analyst salaries in the U.S.
It is estimated that for each mid-sized institution, the average annual total labor costs were about $20.5 million per organization. While traditional rule-based systems and machine learning models exist, they’ve largely failed to solve the fundamental challenges of AML efficiency and effectiveness.
Consilient is changing this through Federated Learning technology, a branch of machine learning. Federated Learning is a novel approach that enables institutions to share behavioral patterns while maintaining data privacy. This is a significant step forward, helping banks detect and prevent financial crime far more efficiently.
This post explains why Federated Learning represents the future of AML compliance and how it’s already delivering results for forward-thinking institutions.
Current challenges in AML risk management
A number of factors in recent years have brought anti-money laundering to the forefront for financial institutions. But there are several critical obstacles in the fight against money laundering:
Manual process inefficiencies 🖱
Manual review of suspicious activity flags requires thousands of analysts yet still fails to deliver comprehensive coverage. The sheer volume of daily transactions makes human-only reviews both impractical and unreliable.
High costs of compliance
The numbers speak for themselves: it is estimated the labor costs for Anti-Money Laundering (AML) compliance are a substantial component of financial crime compliance expenses in the U.S. Labor-related costs typically account for a significant share of the total compliance budget, suspicious activity monitoring, and regulatory reporting.
In 2022, the total cost of financial crime compliance in the U.S. was estimated at $45.9 billion, with a considerable portion attributed to labor.
According to bankdirector.com, U.S. institutions spend $180 million annually on analyst salaries for SAR preparation alone. These mounting costs strain operational budgets and deliver questionable ROI.
Limitations of existing technology ❌
Traditional rigid rules-based systems and same-company machine learning (ML) models aren’t solving the problem. Even large banks that have invested heavily in ML technology struggle to improve detection rates, while their models fall behind ever-evolving money laundering techniques.
Data sharing barriers 🔀
Privacy regulations and security concerns keep valuable insights trapped within individual institutions. Traditional data-sharing methods pose too much risk, while the need to protect proprietary information prevents effective collaboration. The result? Banks fight financial crime in isolation, missing crucial patterns that only emerge when data is analyzed across institutions.
Regulatory pressures 🔍
Financial institutions face increasing scrutiny and potential fines for AML failures, as evidenced by the recent Starling Bank case and TD Bank fine. Keeping up with rapidly changing regulations across different jurisdictions remains challenging.
An intense focus on staying compliant with regulatory requirements leads to an emphasis on the regulator requirements rather than creating new approaches to finding financial crime.
Risk management resistance 🛑
AML and risk management professionals are inherently risk-averse, hesitating to adopt new technologies or approaches. This resistance slows the implementation of more effective AML solutions.
Scalability issues 🌎
As financial institutions grow, their AML processes often fail to scale effectively, leading to gaps in coverage. Rapid customer growth and acquisitions can outpace the expansion of AML capabilities, as seen in the Starling Bank case.
These challenges highlight the need for modernization in AML risk management. Traditional methods prove inadequate against sophisticated money laundering schemes and the volume of global financial transactions. Firms need a solution that enables secure collaboration while maintaining data privacy – this is where Federated Learning comes in.
The Consilient approach: AI Federated Learning
Federated Learning is the breakthrough banks have been waiting for. Instead of asking banks to share sensitive data, Consilient’s AI learns from each institution independently and combines only the behaviors that are flagged as potentially suspicious. The result? A powerful collective defense against financial crime that never compromises security.
Here’s how Consilient works:
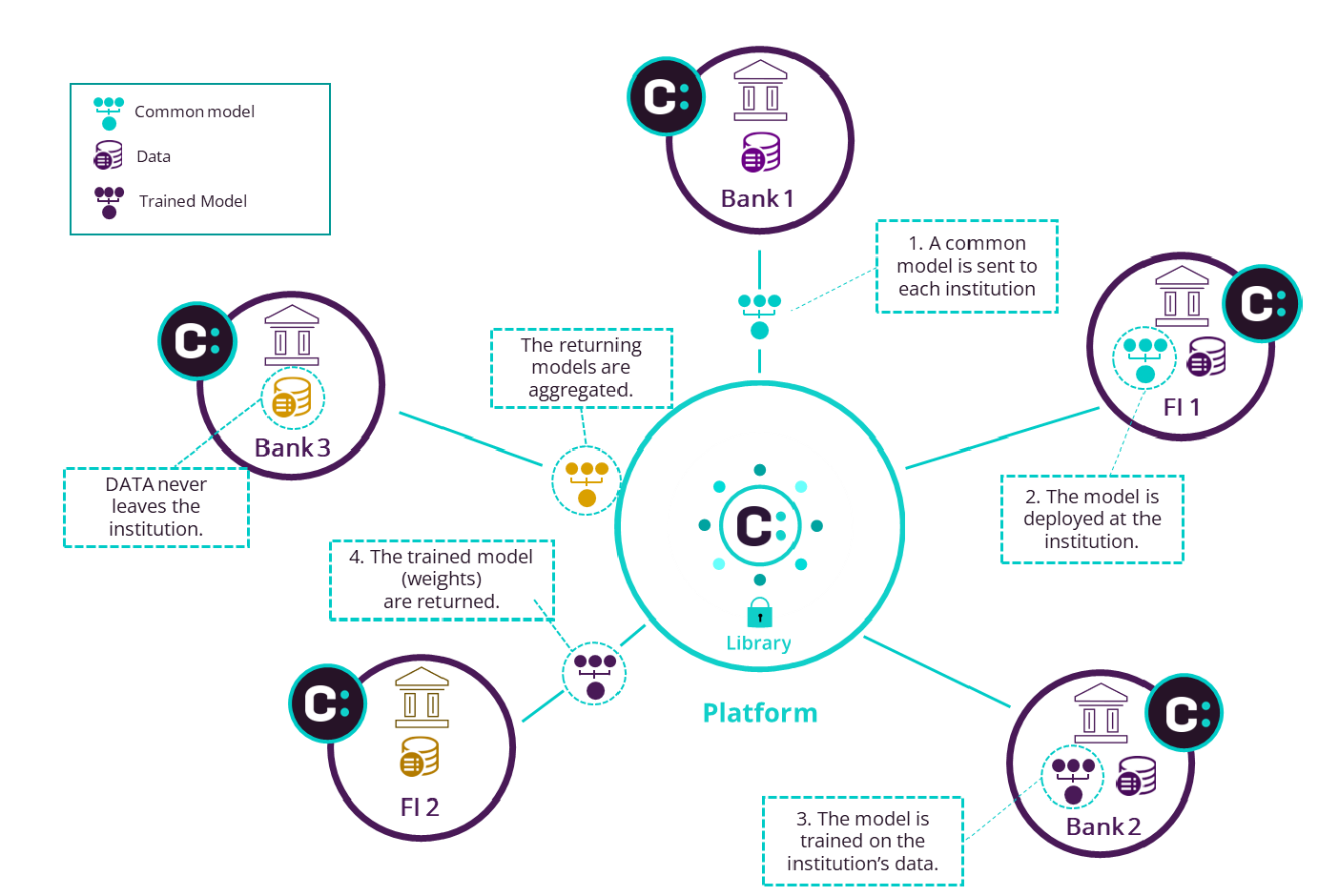
Sharing insights, not data
Our platform moves the model, not your data. The result: Banks gain collective intelligence while maintaining complete control over sensitive information and regulatory compliance.
Enhanced detection capabilities
By learning from multiple institutions, Consilient spots complex behavioral patterns that single-bank models miss. This network effect creates a significantly more powerful defense against financial crime.
Adaptability to emerging threats
Money launderers evolve their tactics daily. Consilient’s AI Federated Learning model updates across the network, providing banks the latest understanding of emerging risks dramatically reducing the gap between new threats and detection.
Proven efficiency gains
Consilient’s technology is proven to be up to 3x more efficient than traditional methods and has detected over 200% more cases in a standard AML transaction monitoring process. This increased efficiency translates to reduced false positives, lower operational costs, and more effective allocation of human resources.
Built-in regulatory compliance
The Federated Learning approach aligns with data protection regulations by design. It enables institutions to collaborate on AML efforts without running afoul of privacy laws or risking data breaches.
Scalability and standardization
Consilient’s solution scales effectively with institutional growth and can standardize risk management across silos in organizations. This ensures consistent AML practices across different branches, subsidiaries, or jurisdictions.
Keen to learn more? Let’s now look at practical use cases.
4 use cases for Consilient’s technology
The benefits of Consilient aren’t theoretical. We’ve seen real results in the banking sector. Here are four ways banks are using our technology today:
#1. Enhanced control: High-risk entity model
Spot high-risk entity behaviors that were previously missed. Our Federated Learning models detect nuanced high-risk patterns by analyzing behavior across multiple institutions, enabling your AML team to focus scrutiny exactly where it’s needed.
#2. Augmentation of existing tools
Consilient layers seamlessly over your current AML systems, enhancing their efficiency and effectiveness without disruption. Institutions can layer advanced insights onto their current systems, improving detection rates and reducing the strain on resources.
#3. Assurance and second line of defense
Consillient’s platform collaborative behavioral insights add a level of verification that mitigates false negatives and enhances the reliability of suspicious activity detection. The result? Institutions can trust that alerts are more accurate, reinforcing their due diligence and helping them stay ahead of evolving financial crime tactics.
#4. Standardized management across organizations
For firms with multiple branches or subsidiaries, maintaining consistent AML practices can be a challenge. Consilient’s platform offers a scalable solution that standardizes risk management across the organization. By enabling consistent, centralized model updates and training across regions, Consilient ensures that all branches benefit from the same high standards, which is especially valuable for institutions operating in diverse regulatory environments.
Why Consilient should become the new AML standard
The case for Federated Learning comes down to five key points:
✅Address the scale of money laundering ($2 trillion annually)
Traditional AML approaches can’t handle the sheer scale of global money laundering. Consilient’s Federated Learning enables banks to fight back collectively, combining insights across institutions to identify and stop illicit flows that no single bank could detect alone.
✅Overcome limitations of current detection rates (<1%)
Current systems catch less than 1% of illicit transactions – despite significant investments in technology and personnel. Consilient’s approach dramatically improves detection by leveraging insights from multiple institutions in real-time, finally giving banks the upper hand against financial crime.
✅Meet regulatory requirements more effectively
With regulators imposing stricter requirements and heavier fines, banks can’t afford AML failures. Consilient’s technology is built for compliance, enabling secure insight sharing while protecting data privacy. The result? More efficient compliance and stronger protection against regulatory risk.
✅Future-proof AML risk management
Financial criminals constantly evolve their tactics. Consilient evolves faster. Our Federated Learning model updates continuously across our network, ensuring your AML defenses stay ahead of emerging threats while scaling seamlessly with your business.
✅The collective approach to prevent money laundering
Consilient leverages the collective intelligence of organizations. The current siloed approach is not going to shift the needle. Consilient brings together behavioral financial crime insights from multiple organizations, creating the ability to draw best practices, knowledge and learning from data together.
The time for better AML is here – and AI Federated Learning is leading the way.
Advancing AML with AI Federated Learning
Consilient brings banks what traditional AML systems can’t: better detection rates without compromising data privacy – bringing a new level of efficiency, accuracy, and adaptability to financial crime detection. It’s a pretty bold statement, but we strongly believe it: This technology is essential in the fight against sophisticated and large-scale money laundering schemes.
Ready to strengthen your AML defenses? Talk to us if you are keen to learn more about Federated Learning. We’d genuinely love to help.
Looking for AML guidance? Take a look at these blogs:
- Lessons from Starling Bank’s AML fine: A wake up call for banks
- From manual to machine learning: The evolution of AML compliance
- How model intelligence is transforming financial crime detection
Source: https://www.bankdirector.com/article/the-high-cost-of-the-suspicious-activity-report/