Why your AML controls should evolve faster than financial crime does
Criminal behavior adapts quickly along with its ability to exploit gaps within AML controls; the moment one detection gap closes, another is found. Transaction Monitoring rules are mostly heuristic and static, and many AML models evolve slowly. If suspicious behavior is detected, action and review are undertaken weeks, if not months, after the event. Companies with machine learning models rely on periodic retraining, static typology libraries, and limited internal data. But that’s no longer enough.
When your systems, rules, models and approaches lag behind how financial crime actually works, the risks are immediate: missed suspicious account activities, misdirected investigators, regulatory pressure, and, worse, a false sense of security.
To keep up, AML systems need to do more than run the same playbook with slight tweaks. They need to learn continuously, adjust to new behaviors across institutions, and evolve without exposing sensitive data. In this blog, we’ll look at what that kind of adaptability really means and why the latest innovative technology—federated models—is becoming essential for institutions that want to stay ahead.
The structural gap between financial crime and detection logic
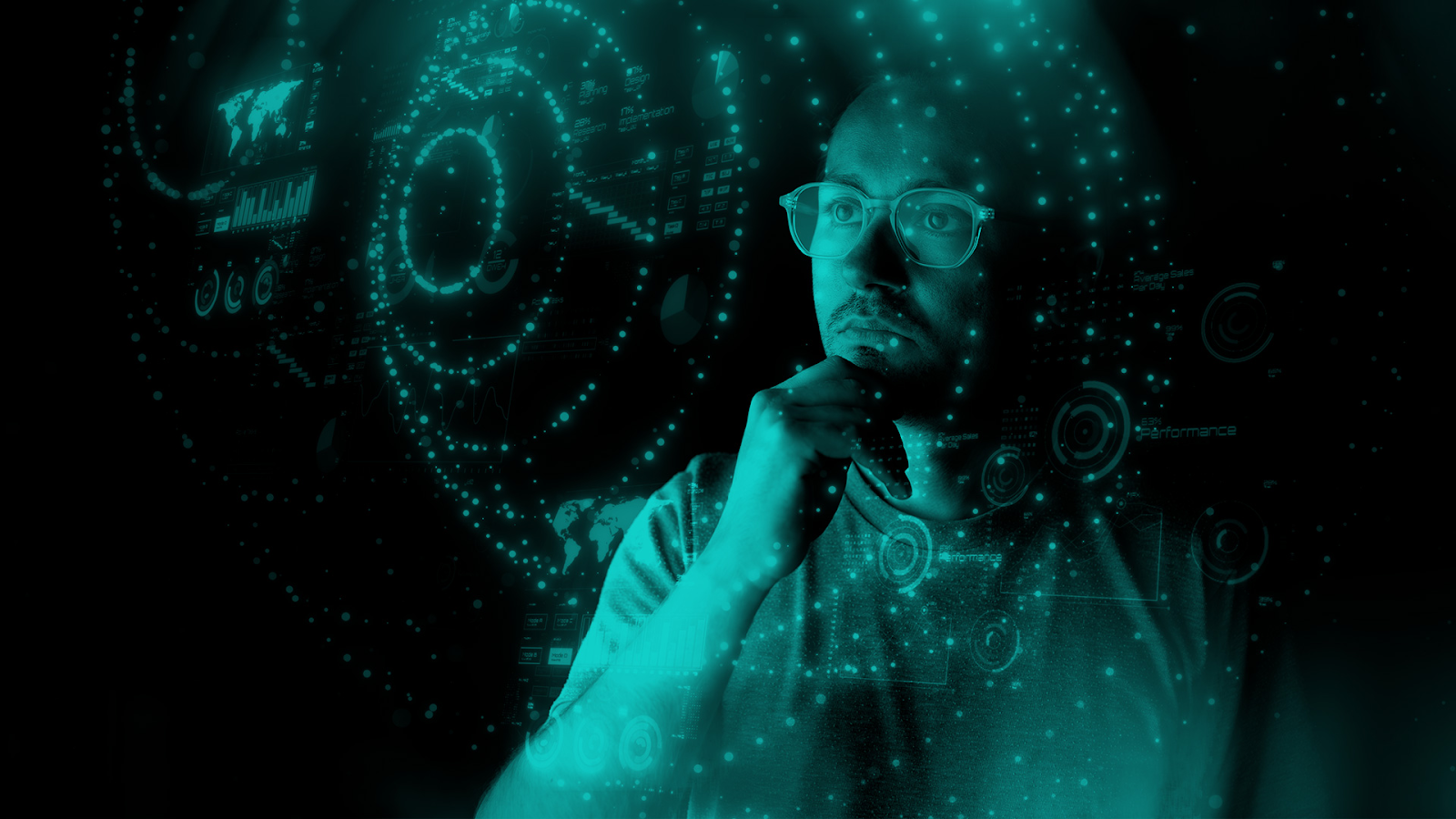
The majority of financial institutions have strong AML compliance teams who take their obligations and roles very seriously. They are, in general, deeply experienced and know what to look for. The real challenge isn’t awareness, it’s an organization’s ability to focus on seeking out and identifying financial crime and balancing this with the enormous task of ensuring that any unusual behavior is reviewed, together with the risks and ability to change and act. Or more specifically, the time it takes to adapt detection systems to new behaviour.
Criminals move quickly, and they understand how to bypass well-known rules. When a regulator changes a requirement, the first to adapt will be money-launderers. Their methods change in weeks, not years. But in many institutions, these changes in behavior are hard to detect, rules are easily evaded, and machine learning model updates miss the new behaviors, as there is limited data to train the models and training is tied to lengthy cycles — governance approvals, validation routines, and technical integration. Even when something suspicious is spotted by a human, feeding that insight back into the system can take months.
Detection logic keeps scoring transactions based on yesterday’s assumptions. Meanwhile, new behaviours, often subtle shifts, not dramatic changes, slide through unnoticed.
That lag creates gaps to be exploited and organizational risk.
The issue is that an organization’s ability to react and update approaches is stymied for multiple reasons and is slow. But speed is often the difference between catching activity early and missing it entirely.
When your controls lag, the risks multiply
When AML controls fall behind and machine learning models are missing new patterns, they miss new risks and compound old ones. Investigators spend more time on irrelevant alerts, leading to teams having to prioritise volume over quality. And senior leaders end up focusing on operational management, regulatory enquiries and lose focus on improving controls to identify new risks and visibility into what the model is actually catching, versus what it’s missing. Over time, that undermines the effectiveness of the entire program.
Then there’s the regulatory angle. Supervisors are increasingly asking institutions to demonstrate how their detection logic is finding risks. And not just through documentation, but through evidence of active improvement. Therein lies the conundrum of defensive filing: the more I file, the better my controls seem to be, proving that an institution is active and proactive. If your controls are static and models haven’t meaningfully changed, and your SAR volumes are unmoved, questions start to follow.
Behind all of that is the reputational risk. When missed activity is discovered by law enforcement, the press, or regulators, the damage includes a loss of trust and material financial pain. In the case of TD Bank, their share price reduced by a massive 9% in the two days following the announcement of their AML fine. Inefficient controls and lagging detection create blind spots, which, at scale, become a headline risk.
All of this can happen quietly, over time. Transaction Monitoring rules are degrading from the time they go live, and a model that once performed well gradually slips out of sync with current behaviours. The alert volumes stay high, but the signal weakens. Even if there is a system in place to track, test and adjust that logic regularly, only being able to utilise outcomes from the existing systems means that controls don’t improve because they are caught in a self-reinforcing loop, the institution is left reacting after the fact, when it’s already too late.
What makes AML controls truly adaptive?
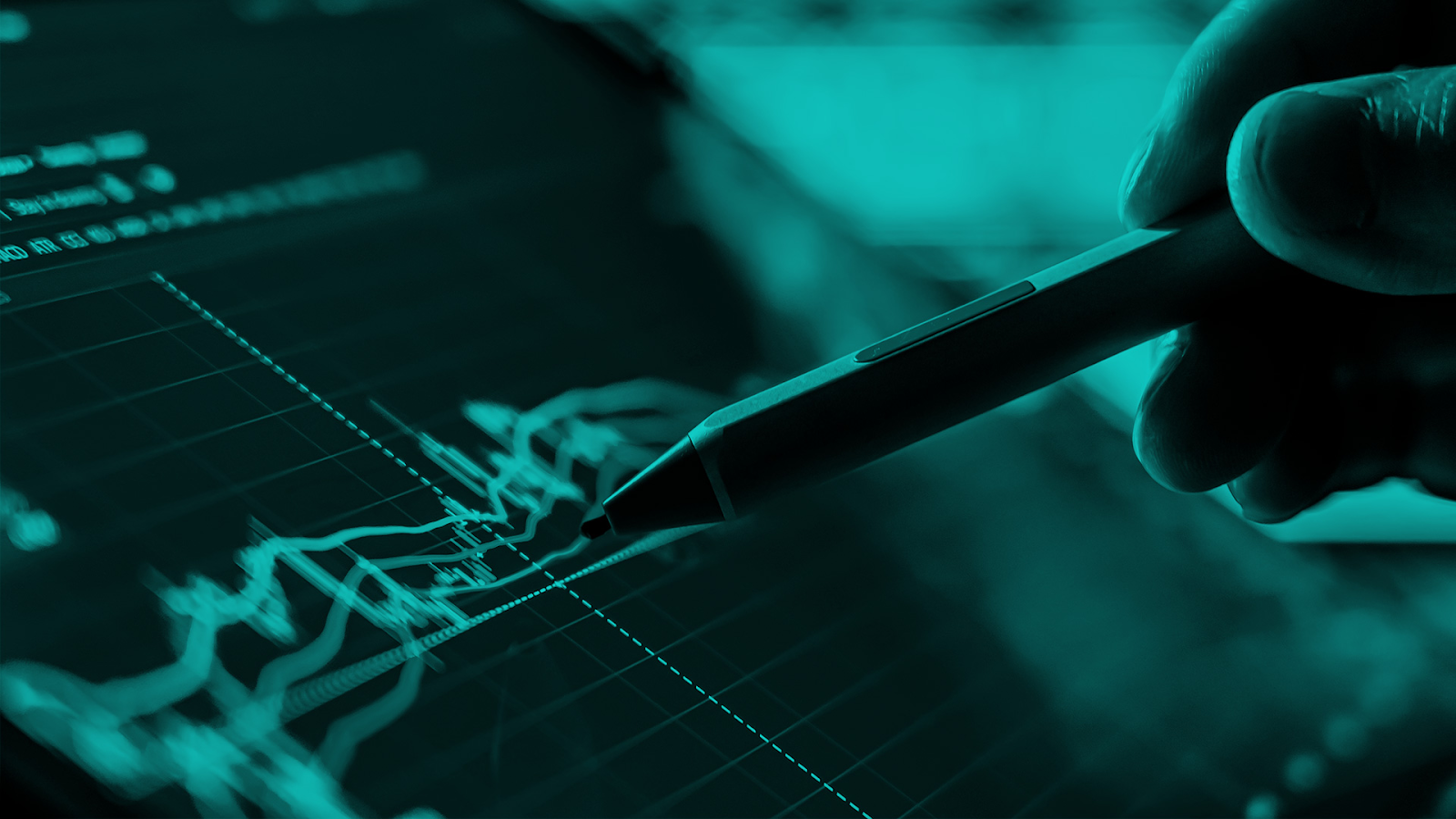
Most institutions would say their controls and models evolve. But in practice, evolution often means waiting for the next retraining cycle, or responding to regulator feedback.
Whereas a truly adaptive model improves continuously, based on how risks develop in real time. And that adaptability depends on a few key things:
1. Ongoing feedback loops
To be effective, controls and models need to learn at the same speed as criminals adjust their approaches. However, as we have identified, due to the enormous volumes of alerts, thereby delaying investigations and case outcome feedback, the need to ensure that an institution’s activity is high, creating the challenge of finding the proverbial needle in the haystack, approaches are missing activity and lagging in efficacy. The loop between front-line review, control updates, and model behaviour should be tight, traceable, and fast.
2. Effective models require access to broader behavioural patterns
Relying solely on internal feedback and data will limit a machine learning model’s ability to spot uncommon or emerging behaviors. The signal is too narrow. Adaptive models need exposure to a wider range of anonymised patterns, especially those that don’t follow behaviors. Internal approaches don’t evolve because they are self-referential. This happens when a system evaluates and adapts itself based only on internal feedback, rather than external benchmarks, so improvements are limited by the system’s own biases or blind spot and this leads to model blindness as the organization or system keeps optimizing based on the same model or assumptions, unaware that the model itself may be flawed or incomplete.
Access to new insights and different patterns is crucial for model efficacy (especially in learning systems, AI, or human decision-making models) for several key reasons:
🟣 Breaks the feedback loop: New insights disrupt the self-reinforcing loop, offering alternative perspectives that can challenge and improve the model.
🟣 Expands the learning space: Models improve by generalizing from diverse experiences. Access to different patterns:
➡Increases variability in training data
➡Helps identify edge cases or exceptions
➡Prevents overfitting to familiar scenarios
Without this diversity, the model learns a narrow worldview and performs poorly on anything outside it.
🟣 Prevents model stagnation: Injecting new patterns helps the model escape local optima and discover more efficient or accurate paths.
🟣 Reduces bias: New insights, especially from underrepresented patterns, help correct systemic blind spots, making the model:
➡More fair
➡More adaptable
➡Better at representing complex real-world variation
🟣 Improves generalization and robustness: A model trained on a wide range of patterns is more flexible, more resilient to anomalies and better at performing in unseen or shifting ecospheres.
3. Detection logic that can evolve without manual rewriting
To ensure AML controls remain agile and responsive to evolving threats, institutions should implement detection logic capable of self-adjustment without requiring manual code changes or developer intervention. Traditional rule-based systems, which rely on frequent updates and formal change control processes, limit the speed and effectiveness of risk detection. If every update requires developer time and change control, agility breaks down.
Adaptive systems need mechanisms for learning at the edge — recognising changes, testing against new data, and refining thresholds.
Manual rules are reactive and can be slow. Self-evolving detection logic can anticipate and respond faster to emerging threats, often before risks become incidents.
4. Built-in explainability
Model adaptability is only useful if it can be explained and justified. Without clear reasoning behind changes in detection, regulators and internal stakeholders will push back.
It’s a tall order, but necessary for firms to keep detection aligned with risk as it evolves, so they can respond with confidence and clarity. Here’s where federated machine learning comes in…
Why federated learning addresses faster, safer control
Federated Learning (FL) solves a major issue in financial crime prevention: how to share intelligence across institutions without sharing sensitive data. As discussed, financial crime patterns evolve quickly, but the ability to learn from those patterns is limited by privacy, regulation, and data silos. Federated Learning breaks that deadlock.
Here’s why it’s different ⬇️
Adapting an AML machine learning model quickly has always been a challenge. Essentially, most institutions work with limited visibility, and internal AML controls and signals can be limited.
Money laundering is rare, and most alerts are false positives. The majority of alerts do not result in SARs or confirmed laundering, creating a severe class imbalance — the model has very few “true” laundering cases to learn from.
And then there’s human decision bias. Outcomes (e.g., SAR filed or not) are based on analyst judgment, not objective truth. Two investigators might reach different conclusions on the same case. Outcomes can reflect process, policy, or resource constraints (not actual risk). Models trained on these may learn human patterns, not laundering patterns.
So understanding what’s happening outside your four walls, what others are finding from their controls, is a completely new way to tackle AML. However, that’s much harder to see, and even harder to learn from.
Federated learning changes that.
It provides institutions the ability to collaborate to identify financial crime. It allows machine learning models to be trained on shared behavioral patterns across institutions without moving or exposing any raw data. The data stays where it is, and only the model learns.
Key advantages for AML teams:
✅Wider behavioral insight: Access to broader, more diverse patterns that improve detection accuracy, particularly for emerging or rare behaviours.
✅It accelerates the detection of emerging threats:
➡Criminals exploit gaps between institutions, using multiple banks, jurisdictions, or accounts.
➡FL can detect cross-institutional laundering patterns (like layering or mule networks) faster than siloed models.
You’re not just learning from your own backyard. You’re seeing the whole neighborhood without invading privacy.
✅It adapts faster to novel behaviors: Traditional models train slowly, and they need central data pulls, cleaning, and governance hoops. Federated models can improve more frequently without the need for a full rebuild or governance-heavy retraining cycle.
➡FL lets models update incrementally at the edge, adapting to new risks in real time and feeding improvements back to the global model.
✅It enables collaborative learning without data sharing:
➡Banks and FIs can train a shared model across multiple organizations without exchanging raw data.
➡Each institution keeps customer data private, but the model learns from trends across all of them.
That means detection logic gets smarter from global exposure — not just local patterns.
What this looks like in practice
Federated learning AML models are already live, tested, and outperforming traditional systems. Institutions using them are improving detection rates while reducing false positives, without adding operational risk or complexity.
Let’s compare traditional methods vs federated learning, side-by-side:
Traditional ML models | Federated Learning | |
Data location | Centralized within a single institution | Distributed across multiple institutions |
Model approach | Data moves to the model | Model moves to the data |
Scope | Limited to patterns within one organization’s dataset | Detects broader patterns across multiple organizations |
Data sharing | Requires sharing of raw data or limited to your own data | No data is shared; only model insights |
Privacy | Potential privacy concerns | Maintains data privacy and security |
Collaboration | Limited or no inter-institutional collaboration | Enables secure collaboration across institutions |
Insight breadth | Constrained by single institution’s data | Benefits from diverse, multi-institutional data |
This is a step change. But not in the data you have — in what your model can do with it.
From lagging to leading: What modern AML needs
AML controls and models can no longer afford to operate in slow cycles. Threats are evolving too fast, and the operational cost of falling behind is growing.
If your detection logic still depends on human-only feedback, scheduled model retraining, static typologies and behaviors, or internal data alone, you’re working with a limited view and a delayed response.
What’s needed now is:
➡Faster learning cycles that respond to change in real time
➡Broader signal exposure beyond your own institution
➡Built-in privacy protection that doesn’t block collaboration
➡Regulatory transparency around how models evolve and perform
Federated learning enables all of this. It unlocks faster control improvement without requiring data sharing or full system overhauls, giving institutions the ability to evolve as quickly as the risks they’re trying to stop. Ready to modernize your AML strategy? Talk to Consilient about how federated learning can help you evolve faster, detect more, and reduce operational strain — all while keeping your data protected.