AI vs AML Compliance: 6 questions every firm should be asking
What happens when your AI moves faster than your compliance team can follow? Unfortunately, this is an actual risk and it’s leaving a huge gap.
As firms push forward with AI to sharpen detection, reduce false positives, and uncover patterns humans would miss, there’s a growing pressure to ensure these tools stay aligned with growing expectations from regulators, auditors, and internal governance teams. But most compliance functions weren’t built to manage machine learning models, and move at the speed these models can develop.
The danger isn’t that AI is too advanced. It’s that the frameworks around it aren’t built to keep pace. And in AML, where transparency, auditability, and trust are non-negotiable, that gap can open the door to significant exposure.
In this blog, we cover what that gap looks like in practice, why current AI tools aren’t always as flexible as they seem and what it takes to build systems that can evolve without stepping outside the lines.
Q1. Why are traditional compliance frameworks failing to keep up?
AI doesn’t wait. Regulators and regulation does.
That’s the tension compliance teams are facing: models that update themselves quickly, and in unsupervised learning in real time, while regulatory expectations shift slowly and often reactively. Rightly regulators are methodical and thoughtful, which creates conflict challenges (1) satisfying regulatory expectations; (2) the need to adapt quickly to utilize the latest technology; and (3) ensuring that frameworks are appropriate and comprehensive to manage the challenge. It’s not that firms aren’t trying to keep up. It’s that they’re building on governance structures designed for static rules, not adaptive systems.
This creates a risk profile most compliance frameworks weren’t built to handle. When your approaches and models change faster than your policies, assurance and compliance teams are challenged to deliver. You might not be able to explain decisions. You might struggle to audit processes. And in AML, where justification is everything, that’s a problem.
Of course, innovation doesn’t need to slow down change. But compliance has to be built in from the start. That means building AI systems that are explainable, auditable, and transparent by design. Not just to satisfy regulators, but to stay in control.
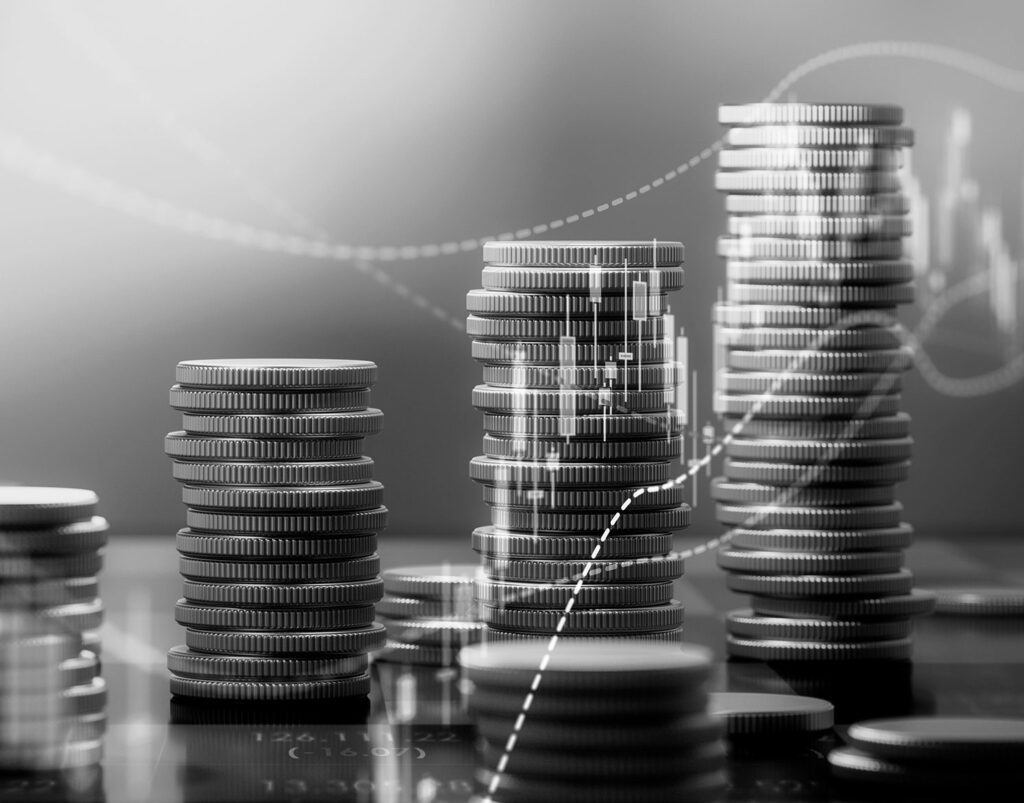
Q2. What’s the risk if your AI can’t explain itself?
If a model can’t explain why it flagged a transaction—or worse, why it didn’t—then no matter how advanced it is, it becomes a liability. Regulators expect clarity. Internal audit teams expect a paper trail. And customers expect fair, consistent treatment. When you can’t deliver that, trust erodes (internally and externally).
That’s what makes the compliance gap so dangerous. It’s not just about fines or findings. It’s about confidence in the system itself. But a question that also needs to be considered is: what is the trust, by users, in the existing legacy systems? Mass false positives can really only mean that investigators and analysts cannot really trust existing systems. But this paradox of trust in old systems vs new is rarely considered.
Think of a flagged transaction that gets escalated. If the model’s logic can’t be traced or justified, what happens in an audit? Multiply that by hundreds of cases per month, and the operational risk becomes systemic. This is equally true of existing systems, not just AI.
For AI, regular tuning and retraining are essential for aligning models with evolving AML risks and regulatory expectations. One of the real positives of using AI is that they are more flexible and can be updated and in fact can be more explainable as they are dynamic. However, they only work if the rest of your infrastructure—data flows, oversight, documentation—can flex with them.
If your AI can evolve but your controls can’t, you’re still behind.
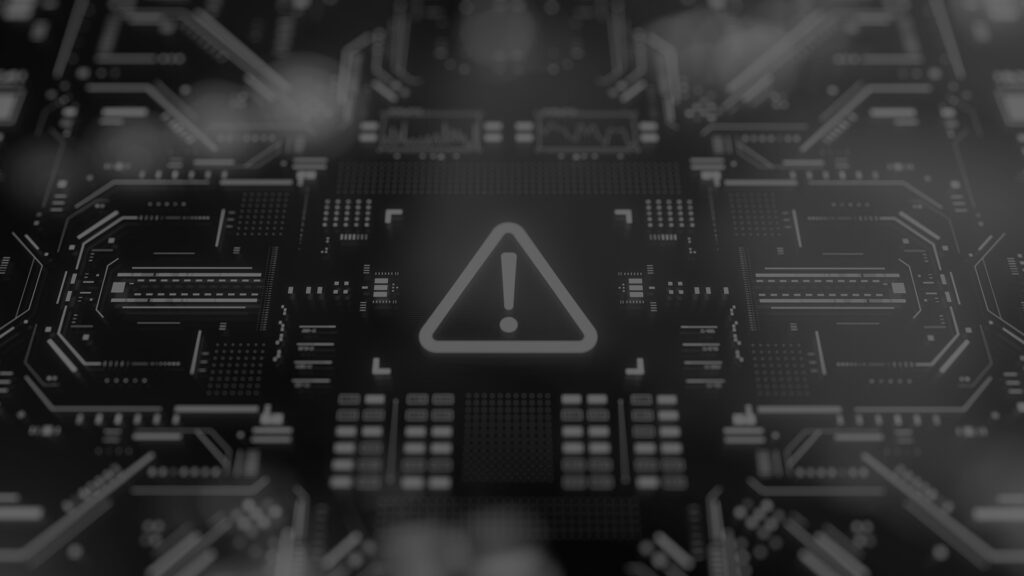
Q3. Can compliance and innovation really move in sync?
Too many firms are still treating compliance as something that happens after the model is built. That approach doesn’t hold up anymore.
AI in AML isn’t static. It evolves with new data, new risks, and shifting regulatory priorities. If compliance teams are reviewing outputs months later, they’re already out of sync.
The fix is structural. Compliance and tech teams need to work side by side, right from the development stage. That means embedding regulatory change management into the AI lifecycle, and including model updates in relevant departmental cadences, so as to not delay launch and compliance functions, circling back to it post-launch.
Typologies change. Sanctions lists update. Guidance from the FinCEN,or local regulators moves on. Your AI needs to adapt in real-time and your compliance controls need to move with it.
If your teams aren’t building together, they’re building gaps.
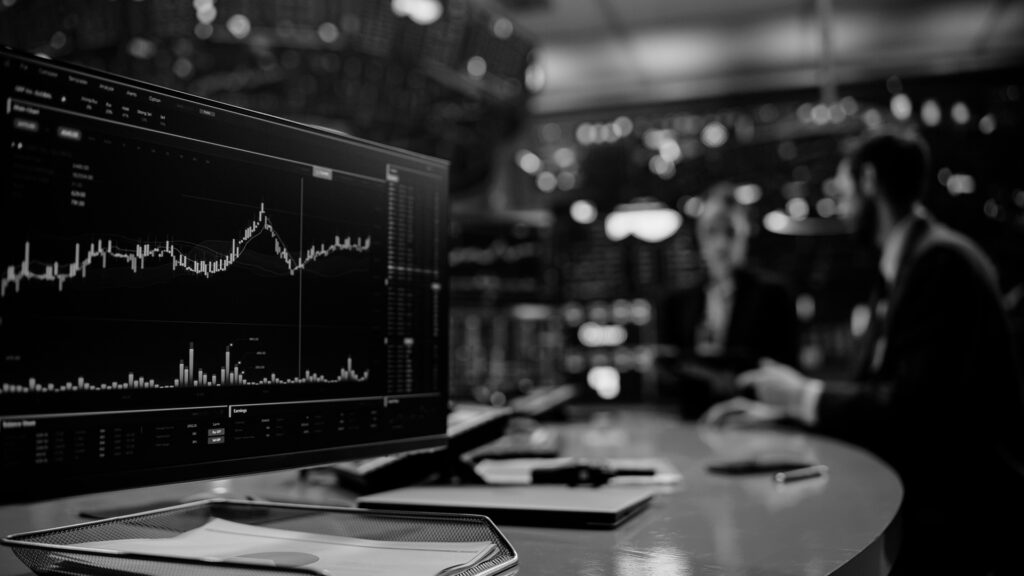
Q4. Are your systems actually flexible? Or is it just your model?
AI is designed to learn. But that flexibility doesn’t count for much if the environment around it is rigid.
In AML, you need to spot suspicious activity in a way that stands up to scrutiny through audits, governance reviews, and regulatory inspections. We don’t just mean the model. It’s everything that surrounds it: data architecture, documentation, oversight, escalation paths.
A flexible model won’t help if your governance layers can’t accommodate change. Without adaptable controls, every model update becomes a compliance risk.
That’s why meeting compliance standards means thinking beyond the model itself. You need systems that support regular tuning, retraining, and risk realignment. Not just technically, but operationally.
This means that inter-departmental functions need to effectively communicate and operate in a highly coordinated way.
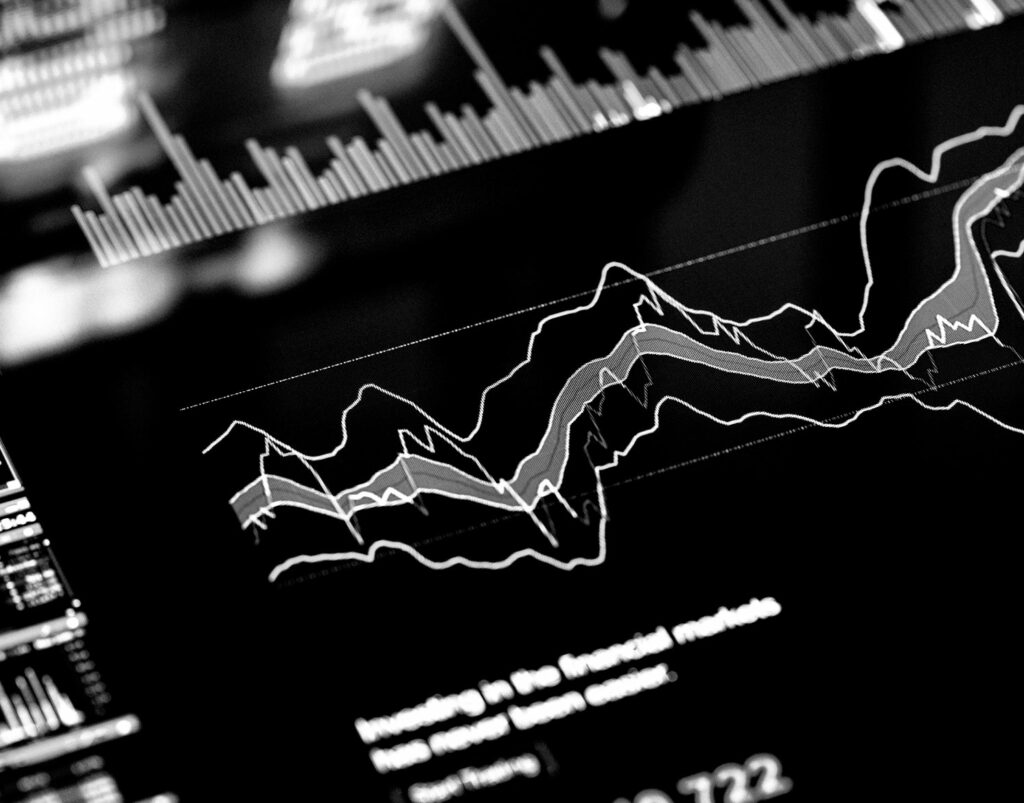
Q5. Should you really bring your AI strategy to regulators?
Plenty of firms are still hesitant to bring AI into regulatory conversations. The fear? That admitting uncertainty will open the door to scrutiny.
But today, silence looks worse. Regulators know AI is evolving. What they want to see is control: clear evidence that firms understand the risks and are building with transparency, accountability, and adaptability in mind.
Some financial institutions are already engaging directly—sharing governance frameworks, participating in working groups, and helping to shape guidance as it develops. Regulators aren’t expecting perfection. But they are looking for proof that you understand how your AI behaves and how it’s being governed. And that you are now looking at the latest technology to assist in your AML/CFT efforts.
Engaging early builds trust. More importantly, it gives you a seat at the table when the next round of guidance is written.
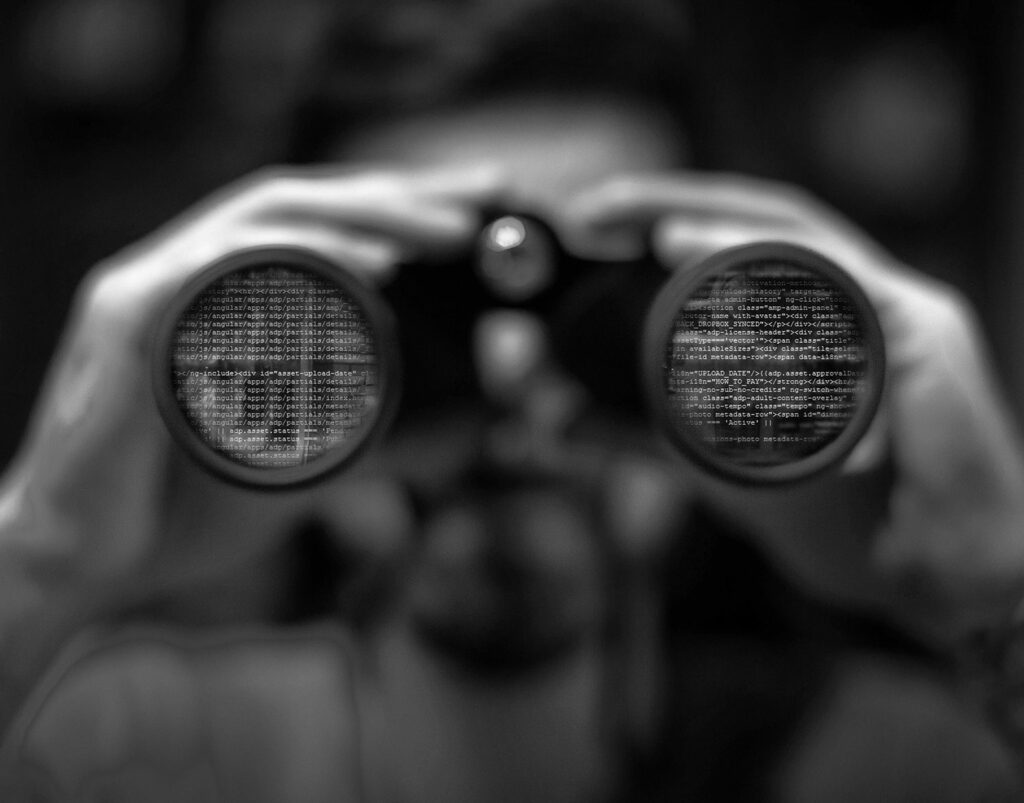
Q6. What does it take to build AI you can stand behind?
AI can absolutely meet the demands of modern AML compliance. But only if it’s built on more than technical performance.
Explainability, auditability, and governance are the foundations. And they need to be in place before your models go live, not retrofitted after the fact.
The firms that will win here aren’t the ones moving fastest. They’re the ones moving with control and engineering systems that can evolve without breaking the rules, and aligning compliance with innovation from day one.
If your AI can’t evolve with regulation, it will eventually break against it.
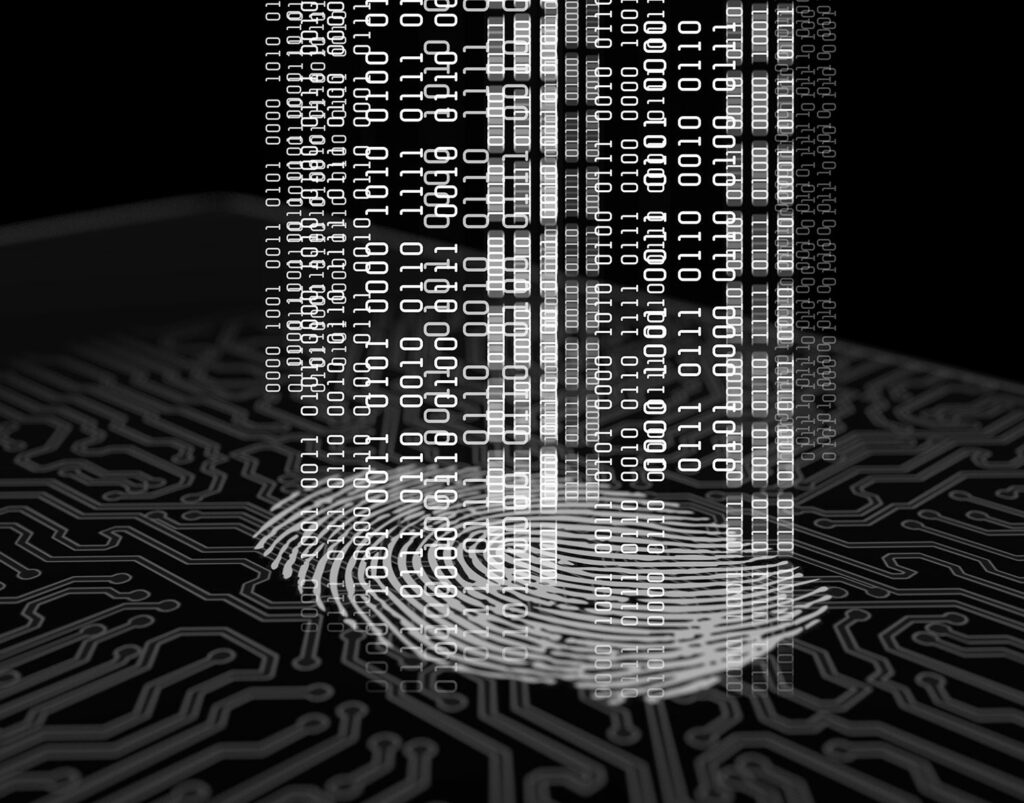
Bringing AI and compliance into alignment
Too many AML tools promise innovation, some make the existing efforts slightly better, but newer advanced technology can leave compliance teams scrambling to keep up. At Consilient, we’ve solved that problem by building compliance into the core of our federated learning model, so you can move fast, stay compliant, and adapt to evolving threats in real-time.
Our approach enables financial institutions to share intelligence without sharing data, unlock stronger detection without increasing false positives, and evolve their systems without risking regulatory fallout.
Don’t take our word for it. We’re recognized in the industry, recently being named in the FinCrimeTech50 2025. One of the top 50 companies leading the transformation in the fight against financial crime. This follows a wave of recognition, having previously been featured in the FinTech100 and named a finalist at EBAday 2025.
We don’t just deliver technology. We help you operationalize it (with explainability, auditability, and governance built-in). That means you can deploy tested and best-in-class AI with the confidence that it’s not only powerful, but regulator-ready.
Whether you’re modernising your existing systems or starting fresh, Consilient helps you close the gap between what your AI can do and what compliance demands. Ready to bring innovation and compliance into sync? Get in touch to learn how we’re helping financial institutions close the gap between innovation and compliance.