Don’t rip and replace: How modern AML models can overlay legacy systems
There’s a reason financial institutions push back on full-scale AML overhauls: they’re expensive, complex, and rarely deliver without disruption. Most legacy systems are deeply embedded in compliance operations and the institution’s risk appetite, governance structure, and reporting obligations. Starting anew isn’t practical, and in many cases, high risk.
Complacency isn’t an option either. Regulatory expectations of compliance teams are rising. While clearly identifying truly suspicious activity rates remain painfully low, there is pressure to improve this performance and reduce costs of doing so at the same time, all without triggering increased regulatory supervision.
The solution? Overlay based-models. By utilizing a supplementary model or logic layer added on top of an existing system, model, or set of rules. It can modify, enhance, or refine the output of the base model.
Utilizing AI to augment and improve existing processes. Machine learning models inject new intelligence into outdated workflows. That means faster detection, more meaningful alerts, and a clearer view of risk, all without dismantling what’s already in place.
This blog explores how modern AI and AML machine learning models, like Consilient’s, can help institutions break through performance ceilings without having to ‘rip and replace’.
The benefits of modern, modular AML overlays
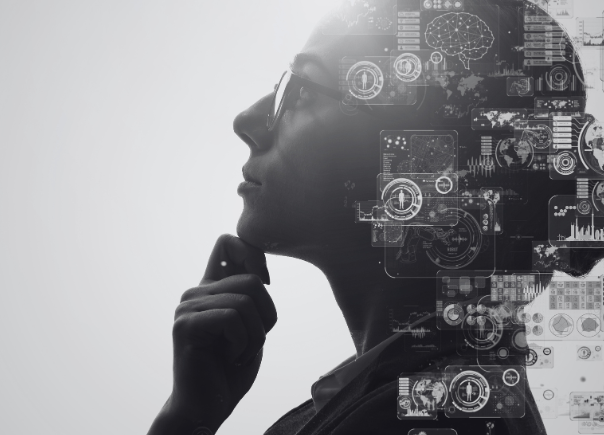
You don’t need to rebuild from the ground up. Overlay-based models offer a more strategic way forward. Instead of competing with your existing systems, they complement them. Designed to slot into your current AML infrastructure, they provide new layers of intelligence that strengthen performance without requiring structural change.
Think of them as an intelligence layer: consuming the same data, interacting with existing case management tools, and more effectively surfacing high-priority risks. Whether it’s smarter alert prioritization, refined scoring, or a second-line review layer, these capabilities lift the ceiling on what legacy systems can achieve.
In fact, some banks and financial institutions are already using overlay models to:
- ✅Surface higher-risk activity faster
- ✅Improve signal-to-noise ratios in alert queues
- ✅Introduce adaptive scoring powered by federated learning
- ✅Reduce manual review pressure on compliance teams
This is what modernization looks like when you stop trying to rip and replace and start building intelligently around what works.
📕Further reading: What banks did wrong in 2024: Critical AML failures and essential lessons
How to accelerate detection without disrupting operations
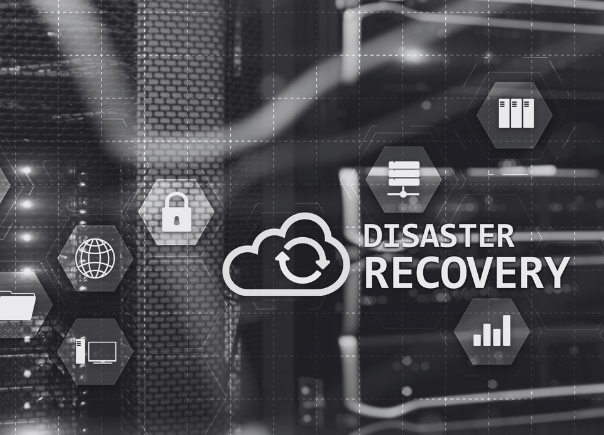
For many institutions, improving AML effectiveness feels like a trade-off: either investing in long, expensive, resource-intensive upgrades that come with significant change risk or accepting existing systems’ limitations. Overlay models challenge that assumption.
Improvement without disruption.
Here’s how:
➡Faster deployment, faster impact
Because overlay models work with existing platforms, they bypass many of the delays that hold back traditional transformation efforts. There’s no need to reengineer core systems, rebuild data pipelines, or migrate workflows. Instead, overlays consume existing data, connect via APIs or batch integrations, and deliver insight directly into current processes.
This accelerates time-to-value. Institutions can transition from pilot to production in a matter of months, dramatically reducing the lag between investment and measurable improvements in detection rates, alert quality, and review efficiency.
➡Improved detection with lower friction
Overlay models also sharpen the institution’s ability to detect and respond to risk. By applying machine learning-based scoring, adaptive prioritisation, and pattern recognition on top of legacy rule sets, overlay models help surface the riskiest behaviours sooner and with fewer false positives.
That means faster triage, smarter investigations, and reduced time wasted on dead-end alerts. It also allows institutions to stay ahead of evolving criminal behaviour without constantly reconfiguring base systems or rules.
➡Operational continuity as a design principle
One of the most overlooked advantages of overlay models is how well they preserve internal stability. AML identification systems don’t exist in isolation—they’re connected to case management, regulatory reporting, internal controls, and audit trails. Replacing them can be deeply disruptive.
Overlay models avoid this. They are built to fit around existing processes, not force new ones. Investigators continue using the tools they know. Regulatory traceability remains intact. Change management becomes incremental, not wholesale.
For institutions under scrutiny from regulators, boards, or audit committees, this ability to improve without upheaval can be the difference between progress and paralysis.
Federated learning: Better outcomes, without sharing data
Consilient’s model takes this one step further with federated machine learning. This privacy-preserving approach enables AML models to learn from broader financial crime patterns across institutions without moving or exposing any customer data.
This enables detection models to benefit from a broader range of typologies, risk signals, and network intelligence, delivering stronger performance than any institution could achieve in isolation. And because all data remains where it is, there is no additional burden on governance, legal, or data protection teams.
The result? A faster, smarter, more collaborative AML.
📕Further reading: Why your AML controls should evolve faster than financial crime does
Use cases: early warning systems, prioritisation scoring, enhanced review layers
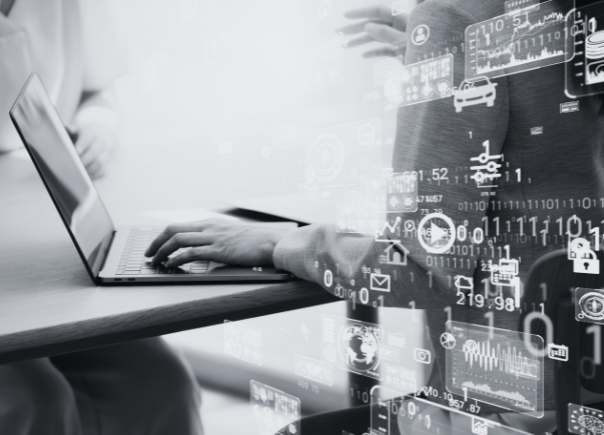
The strength of overlay-based AML models lies in their flexibility. They can be deployed to support targeted pain points, or layered across the entire detection and review lifecycle. Below are some of the most common and high-impact use cases where institutions see measurable gains.
#1. Early warning systems
Overlay models can act as a proactive surveillance layer, surfacing patterns of activity that may not trigger traditional rules, but show elevated risk when viewed in context. This supports earlier intervention, better triage, and reduced exposure to long-tail criminal activity.
With federated learning, these early signals become even more powerful. By learning from high risk behaviors and typologies seen across a network of institutions, the model can identify cases and transactions that might otherwise be missed when viewed in isolation.
#2. Risk-based alert prioritisation
Not all alerts carry the same weight. Yet many legacy systems treat them as if they do, resulting in overwhelmed teams and high false-positive rates. Overlay models help rank alerts by risk, using machine learning models trained on historic outcomes, case attributes, and external patterns.
This supports smarter allocation of investigative resources and increases the chances that true positives are caught early, not buried in the queue.
#3. Enhanced review layers
Overlay models can be deployed as a second layer of review—applying advanced scoring or high risk typology detection after the core system has generated an alert. This gives teams additional context, helps suppress false positives, and supports more confident decision-making.
These enhancements also create a valuable audit trail, demonstrating to regulators that the institution is investing in improving detection quality and effectiveness.
#4. Cross-institutional learning (via federated models)
One of the most powerful applications of overlays is in enabling shared learning across institutions without sharing data. Through federated learning, overlays can benefit from typologies and risk patterns identified elsewhere, strengthening each institution’s model in the process.
This offers a new kind of collective defense: improving performance while maintaining privacy, data sovereignty, and regulatory alignment.
Stronger AML, same infrastructure
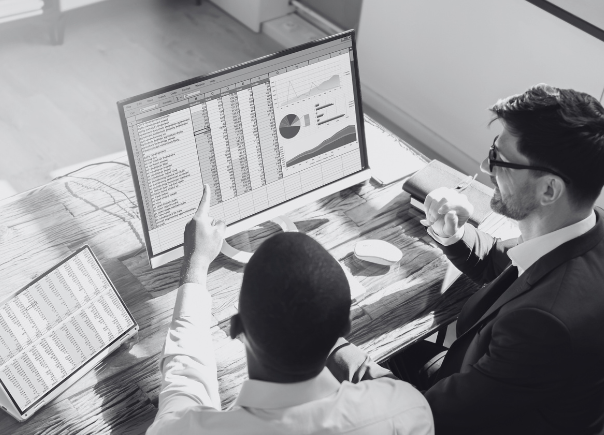
Financial institutions don’t need to rip out the foundations to make meaningful progress in AML. The idea that performance gains require wholesale replacement is outdated—and in many cases, counterproductive. Today’s challenges demand faster responses, stronger intelligence, and a more flexible approach to implementation.
That’s exactly where overlay-based models excel. By building on top of existing systems, they help institutions raise the ceiling on what’s possible—improving detection accuracy, reducing false positives, and giving investigative teams the space to focus on what really matters. And when these models are powered by federated learning, the benefits go even further. Institutions can tap into a wider pool of typologies and shared intelligence, without exposing sensitive data or disrupting internal processes.
This is AML progress that respects institutional reality: complex infrastructures, tight timelines, and rising regulatory pressure. It’s a more efficient way to modernise. And it’s a safer, smarter one.
Ready to move beyond the limitations of legacy systems without starting from scratch? Talk to us about how Consilient’s federated learning model can work with your existing AML framework.