How AI helps governments and businesses tackle AML and economic crime
Tackling money laundering and economic crime remains a significant challenge for governments and businesses. These issues span technology, politics, legal frameworks, and regulation—affecting everyone from regulators and financial intelligence units to banks and financial institutions.
At the heart of the problem lies the need for better data sharing and communication. Yet, strict privacy laws such as GDPR (Europe) and CCPA (California), alongside security concerns and political pressures, often stall progress. Despite these barriers, there is a solution worth exploring: AI.
The public-private disconnect: Why AML suffers
While frameworks like the Egmont Group facilitate some cross-border collaboration, achieving a coordinated, unbiased, and resource-efficient approach remains a significant hurdle. This lack of alignment complicates efforts to combat financial crime effectively.
Of course, this isn’t to suggest that government agencies lack diligence in their governance or intentions. However, inflexible regulations and varying interpretations often create unintended consequences, stifling innovation and burdening institutions with escalating costs.
These challenges cascade to financial institutions tasked with meeting oversight requirements, leading to:
🔍 Competing priorities
While banks focus on risk management and compliance, regulators prioritize oversight, and FIUs concentrate on intelligence gathering. These divergent goals create blind spots that sophisticated criminals exploit.
🚧 Regulatory gridlock
Despite good intentions, current regulations often work against themselves. Banks face steep penalties for compliance missteps, leading to defensive filing of suspicious activity reports (SARs) rather than meaningful risk detection.
💰 Spiraling costs
The numbers are staggering: banks spend an estimated $300 billion annually on financial crime compliance. Yet, detection rates remain below 1%—a clear sign that throwing money at the problem isn’t working.
🔒 Failed collaboration
Previous attempts at data sharing initiatives have stumbled over privacy concerns (such as TMNL in the Netherlands) and institutional mistrust. The result? Valuable intelligence remains trapped in silos.
In the words of Sir Humphrey Appleby (UK TV’s Yes Minister), “The Civil Service thrives on self-generated work. It increases its importance, its influence, and its budget.”
The problem with traditional AML systems
Current transaction monitoring (TM) systems compound these challenges. Here’s why they’re so weak:
⚠️ Drowning in false positives
Traditional systems flag up to 95% false positives, overwhelming investigation teams with dead-end leads while real criminals slip through unnoticed.
📊 Rigid rule sets
Fixed rules can’t adapt to evolving criminal tactics. When criminals change their behavior, these systems fall behind—often by months or years.
💸 Resource drain
Banks spend millions investigating false alerts while missing sophisticated criminal networks. One major bank reported reviewing over 30,000 alerts monthly, with less than 2% leading to actual SARs.
The message is clear: incremental improvements to existing systems won’t solve these fundamental challenges. We need a revolutionary approach to AML that enables secure collaboration while preserving privacy and regulatory compliance.
When AI meets AML: Finally addressing AML challenges
AI has an enormous role to play in resolving the inefficiencies in the AML ecosphere. Its ability to streamline processes and uncover hidden patterns makes it a powerful tool in the fight against financial crime.
Adopting new technology, such as AI, is widely discussed by government agencies and commercial organizations. The right AI models can change, streamline, and improve customer, operational, and business processes. The AML industry, from regulators to financial institutions, is crying out loudly for innovation. Yet, all are stuck with existing systems and processes at enormous expense. Why?
AI often faces skepticism due to its perceived risks. AI can lack the sophistication to operate autonomously in AML activities. AI models can perform poorly when encountering scenarios outside their training data, known as the out-of-distribution problem. At any one organization, AI training is limited to what that organization already knows, and therefore limits its ability to identify changes in money laundering behavior.
They lack adaptability in situations requiring creativity or improvisation beyond programmed logic. AI’s limitations—such as contextual understanding, ethical biases, and creative adaptability—mean it cannot fully replace human expertise in this domain.
Despite these limitations, AI’s strengths make it a critical asset when thoughtfully implemented. Its ability to enhance efficiency and improve the precision of existing processes provides a clear opportunity to modernize AML efforts.
How AI works in AML
AI excels at handling high dimensionality. Techniques like deep learning can process high-dimensional data efficiently, identifying subtle interactions among variables. Machine Learning models learn patterns directly from data without predefined rules. This allows them to identify relationships, trends, and anomalies even in highly complex or noisy datasets far better than traditional approaches.
AI’s ability to deliver value also hinges on its access to data. Data forms the foundation for understanding patterns, making predictions, and generating actionable insights.
AI systems rely on large datasets to train their algorithms. These datasets act as examples, enabling models to identify patterns, relationships, and behaviors. Over time, exposure to new and varied data allows AI systems to evolve, refining their predictions to remain relevant as conditions change.
Diverse datasets are key to maximizing AI’s performance. Broader, more varied data helps models generalize better, reducing biases and improving accuracy across different scenarios. This diversity is critical for tackling complex challenges like identifying nuanced money laundering behaviors.
However, this is where a significant challenge arises. When AI operates in isolated data silos, its ability to learn and improve is limited. This fragmentation creates serious vulnerabilities:
⏺ Money launderers can spread activities across multiple institutions to avoid detection
⏺ AI models develop blind spots from training on limited data
⏺ Banks invest in sophisticated technology but still miss complex criminal networks
⏺ Biased or incomplete training data leads to flawed detection models
To overcome these limitations, AI needs access to collaborative frameworks that preserve privacy while enabling richer, more inclusive training. This is where Federated Learning comes into play.
Why Federated Learning represents a paradigm shift for the AML industry
Federated Learning represents a practical solution for organizations facing the dual challenge of collaboration and privacy. Allowing AI models to learn from multiple data sources without sharing sensitive information addresses the major barriers to AML detection.
Markus Shultz, the Chief Technology Officer of K2 Integrity and former Deputy Global Head of FCC & Head of Change Management for Financial Crimes Compliance (FCC) at ING, states:
“Financial crime is organized and distributed, leaving limited ability for any one unit to see the whole picture. AI and federated machine learning are essential to better identify and combat financial crimes across units and boundaries. Consilient is uniquely positioned with its expertise and solutions to help the private and public sector overcome hurdles, and these boundaries add a superpower to any financial crime fighter.”
Here’s how Federated Learning works in practice:
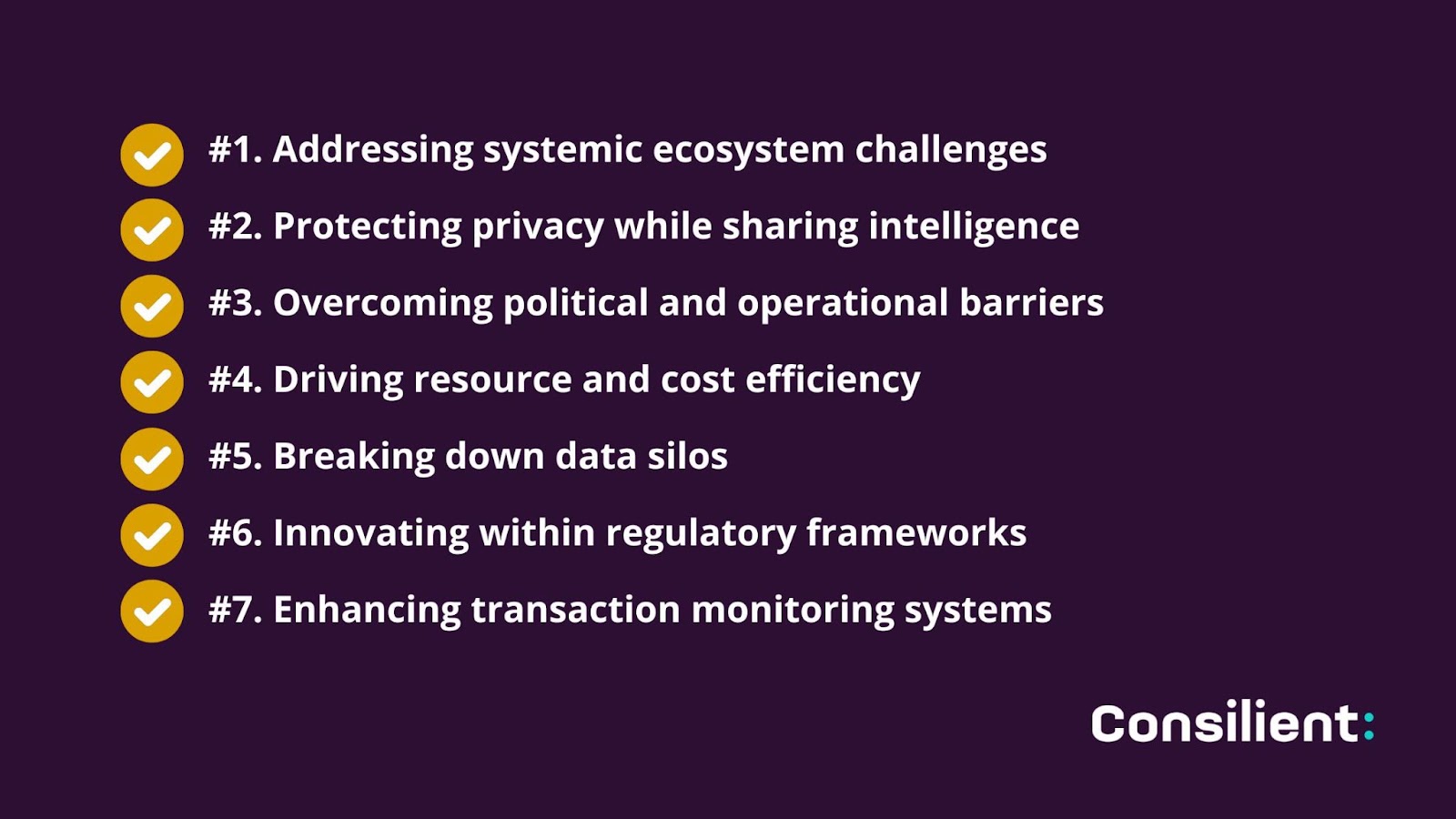
1. Addressing systemic ecosystem challenges
⏺ Unified ecosystem: Federated Learning connects regulators, financial institutions, and other stakeholders, creating collaborative models while respecting independence.
⏺ Agility in policy implementation: Shared models ensure consistent policy application across jurisdictions and institutions.
⏺ Bias mitigation: By incorporating insights from diverse datasets, Federated Learning reduces biases inherent in isolated training environments.
2. Protecting privacy while sharing intelligence
⏺ Decentralized training: Federated Learning trains AI models on local datasets within an organization’s secure infrastructure. Only aggregated model updates—not raw data—are shared.
⏺ Advanced privacy preservation: Techniques like differential privacy and secure multiparty computation ensure compliance with strict privacy regulations.
⏺ Reduced exposure: By avoiding centralized data pooling, Federated Learning minimizes the risk of data breaches and addresses security concerns in inter-agency or inter-institutional collaborations.
3. Overcoming political and operational barriers
⏺ Independent control: Federated learning allows participants to maintain full control over their data while contributing to shared AI models, respecting the need for autonomy.
⏺ Building trust: Transparent governance frameworks enable trust, encouraging collaboration without concerns over data misuse.
⏺ Aligned goals: Federated learning focuses stakeholders on shared outcomes, such as improved detection rates, rather than conflicting priorities.
4. Driving resource and cost efficiency
⏺ Improved detection models: Federated learning-trained models reduce false positives in transaction monitoring (TM) systems, decreasing the strain of unnecessary investigations.
⏺ Real-time updates: Continuous learning and refinement of AI models improve detection capabilities without requiring costly system overhauls.
5. Breaking down data silos
⏺ Collaborative insight: Federated Learning aggregates knowledge from diverse institutions, such as banks and regulators, without compromising data locality. This enhances the detection of global ML typologies.
⏺ Diverse dataset utilization: Insights from various data sources create more generalized AI models capable of identifying nuanced criminal behaviors.
⏺ Cross-jurisdictional analysis: Federated Learning bridges geographic and regulatory divides, enabling detection of cross-border ML schemes while respecting local data laws.
6. Innovating within regulatory frameworks
⏺ Compliance-driven innovation: Federated Learning adheres to existing privacy laws, offering a regulatory-friendly solution that minimizes resistance to adoption.
⏺ Scalable solutions: Its decentralized architecture accommodates organizations of all sizes without disrupting existing processes.
7. Enhancing transaction monitoring systems
⏺ Advanced pattern recognition: Federated Learning-trained models leverage a broader data spectrum to detect subtle and complex ML patterns.
⏺ Dynamic risk assessment: Regular updates with new data ensure models stay relevant to emerging threats.
⏺ Reduced False Positives: Federated Learning creates more precise models, distinguishing legitimate activity from genuine risks, and improving the accuracy of flagged transactions.
By bridging gaps in collaboration, privacy, and technology, Federated Learning empowers stakeholders across the AML ecosystem to work smarter, reduce inefficiencies, and stay ahead of increasingly complex financial crime. You know what makes this even better? Our new Core AML/CFT model can detect these patterns while maintaining complete data privacy. See the details here.
A coordinated future with Federated Learning
Federated learning provides a practical, innovative solution to combating money laundering and economic crime. It preserves data privacy, promotes collaboration, reduces costs, and enhances the effectiveness of AI in detecting and preventing illicit activities. FL paves the way for a unified, efficient, and impactful AML ecosystem by addressing technological, regulatory, and operational barriers.
Ready to strengthen your AML program? Let’s talk about how federated learning can help you catch more criminals while reducing costs. Contact us today.
Further reading:
📕Anomaly detection at scale: Consilient’s approach to high-risk entities
📕Banking on collaboration: How to overcome the stalemate in AML innovation
📕Anti-money laundering (AML) risk management reimagined: The Consilient approach