It’s time to look beyond traditional Transaction Monitoring systems for AML
Traditional transaction monitoring (TM) systems are failing. Designed to catch suspicious activity and keep banks compliant, these systems have become a costly burden that criminals easily outsmart.
The reality is stark: TM systems overwhelm compliance teams with false positives while sophisticated criminals slip through undetected. Banks sink millions into rigid, rules-based systems that can’t adapt to evolving money laundering tactics. The result? A reactive approach to financial crime that leaves institutions perpetually playing catch-up.
This isn’t just inefficient—it’s dangerous. Money launderers know exactly how to exploit these static systems, spreading their activities across multiple institutions to avoid detection. Meanwhile, compliance teams waste countless hours investigating legitimate transactions that their TM systems wrongly flag as suspicious.
In this post, we’ll expose the critical flaws in traditional TM systems, examine why banks remain tethered to these outdated tools, and reveal how advanced AML/CFT models offer a way forward.
Why traditional Transaction Monitoring is failing banks
Legacy TM systems aren’t just inefficient—they’re actively holding banks back. Here’s what’s going wrong:
🚨False positives overload
TM systems rely on fixed, rule-based models to flag suspicious activity. However, these rules often lack the nuance to distinguish between legitimate transactions and genuinely suspicious behavior. For example:
🟣High-volume businesses, cross-border transactions, or complex customer profiles are frequently flagged as risky, even when the activity is routine.
🟣Compliance teams are left sifting through thousands of alerts, with up to 95% of flagged transactions proving to be false positives.
This not only wastes resources but also diverts attention from genuinely high-risk cases, undermining the effectiveness of AML efforts.
🚨Wide risk coverage at the expense of precision
To comply with regulatory expectations, institutions often err on the side of caution by implementing broad, rigid rules. While this approach reduces the likelihood of missing potential money laundering cases, it comes with significant trade-offs:
🟣Nearly all deviations from predefined “normal” behavior are flagged as suspicious, even when they pose no real risk.
🟣This dilutes focus from high-priority threats, creating inefficiencies and burdening compliance teams with unnecessary investigations.
🚨Inability to adapt to evolving threats
While criminals constantly evolve their tactics, TM systems remain frozen in time. Fixed-rule TM systems struggle to adapt to these emerging typologies, leaving institutions vulnerable to:
🟣New laundering methods such as the use of mule accounts or cryptocurrency transactions.
🟣Loopholes that static systems cannot anticipate or address without manual intervention.
🚨Operational and reputational costs
False positives aren’t just an operational headache—they’re destroying value. Legitimate transactions can be delayed, customer accounts incorrectly flagged, and trust eroded. Over time, these issues result in:
🟣Damaged customer relationships and reduced retention.
🟣Tarnished reputations for financial institutions, which may be seen as inefficient or overly intrusive.
🚨Missed opportunities for proactive detection
Traditional TM systems focus narrowly on predefined rules and behaviors, missing the opportunity to leverage advanced technologies like AI and machine learning. These technologies could:
🟣Dynamically analyze transaction patterns to refine detection accuracy.
🟣Reduce false positives while uncovering hidden risks.
The message is clear: banks can’t afford to keep using systems that catch less than 1% of financial crime while burning through resources on false positives. It’s time for a smarter approach.
The real reasons banks can’t break free from legacy systems
Banks know their TM systems aren’t working well. Yet they stay trapped in expensive, inefficient processes. Here’s why they continue to rely on these outdated systems for several reasons:
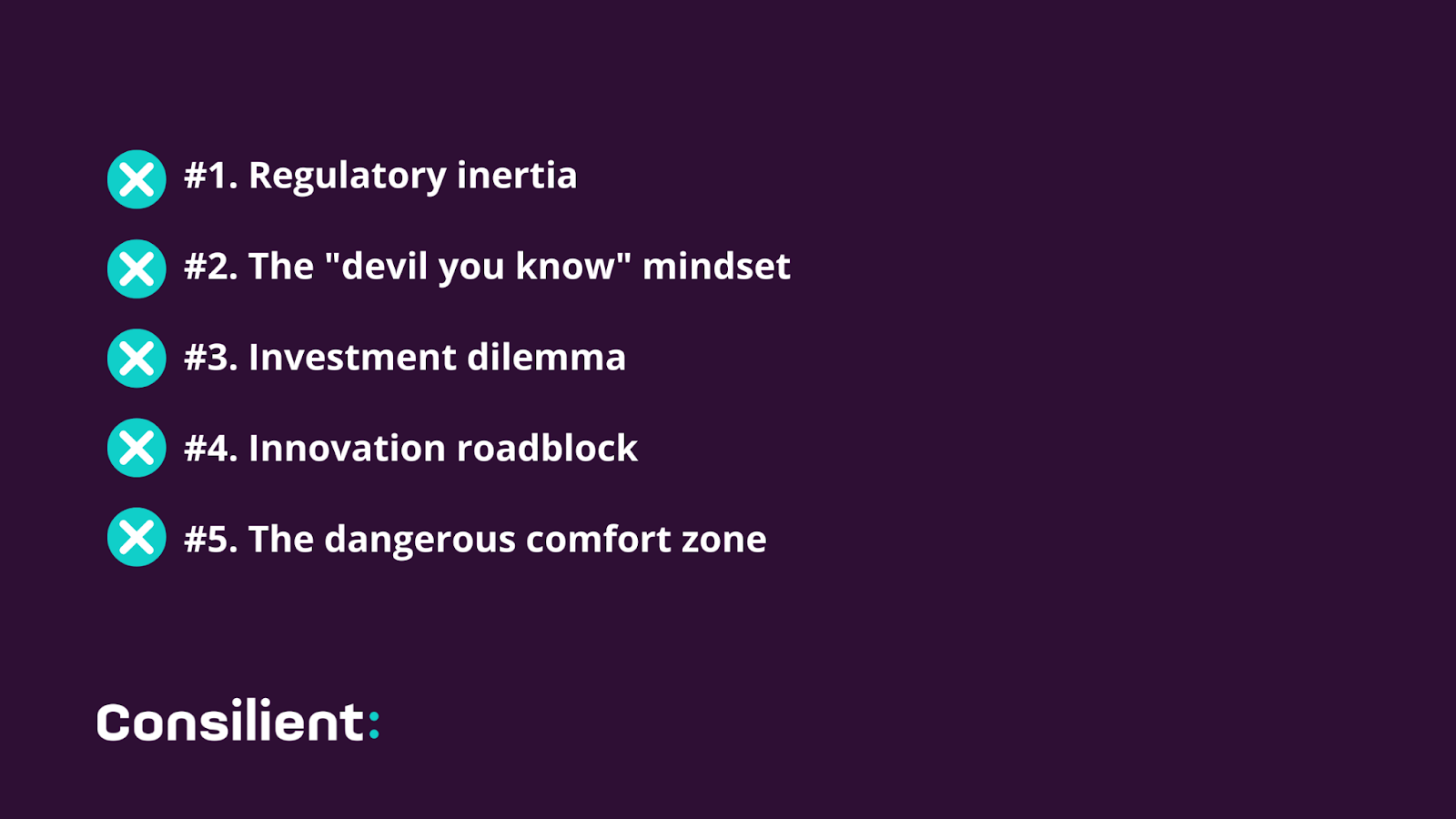
#1. Regulatory inertia
Banks are somewhat paralyzed by compliance fears. Any change to existing systems brings regulatory scrutiny, and the cost of getting it wrong is massive. Financial institutions face steep fines and reputational damage if they misstep. The result? Innovation gets stifled before it starts, as compliance teams choose the safety of outdated systems over the promise of better detection.
#2. The “devil you know” mindset
Replacing legacy systems means disrupting daily operations, potentially compromising compliance, and retraining entire teams. This uncertainty leads banks to stick with familiar failures instead of embracing innovation. It’s a classic case of choosing the known problems over unknown challenges—even when those known problems cost millions.
#3. Investment dilemma
Most banks have sunk millions into their current systems. Making a change means writing off these investments, bearing additional costs of implementation and possibly dual running while funding new technology adoption.
On the face of it, investing in compliance does not drive enterprise and shareholder value. It has been repeatedly proven that a lack of investment in core processes and security can ultimately cost much more than updating out-moded services. For many institutions, especially smaller ones, the initial cost of switching systems creates a significant barrier—even when the long-term savings would justify the investment.
#4. Innovation roadblock
Organizational barriers consistently kill progress. Decision-makers resist solutions they view as unproven, while teams working in silos block effective collaboration. This risk-averse culture, combined with short-term thinking, prevents banks from making changes that could transform their AML effectiveness.
#5. The dangerous comfort zone
Many banks tell themselves their systems are “good enough.” They ignore the millions wasted on false positives, the criminals exploiting known weaknesses, and the growing operational inefficiencies. This misplaced confidence masks the rising cost of maintaining the status quo.
Here’s the truth: staying with legacy systems isn’t the safe choice anymore. While banks hesitate, criminals innovate. Traditional TM systems aren’t just inefficient—they’re becoming dangerous liabilities.
But there’s good news: Federated Learning offers a way forward that doesn’t require ripping out existing systems. In the next section, we’ll show you how.
Real-world impact: How Federated Learning delivers results
Want proof that Federated Learning works? Look at the numbers. Leading banks are already using this technology to transform their AML detection from a cost center into a powerful crime-fighting tool.
Case study: Reducing false positives and improving detection rates
One major financial institution implemented Federated Learning to address its overwhelming volume of false positives. Here’s what they achieved:
⏺75% reduction in false positives: Compliance teams could focus their resources on truly suspicious activity, improving efficiency and response times.
⏺300% increase in detection rates: By leveraging insights from collaborative AI models, the institution uncovered hidden patterns missed by their legacy TM system.
⏺Improved regulatory relationships: Enhanced precision and transparency built trust with regulators, reducing compliance risks and penalties.
For the first time, banks collaborate to catch criminals without exposing sensitive data. Here’s what this looks like in practice:
🟣Institutions shared suspicious activity patterns (not raw data) to identify cross-border money laundering typologies.
🟣Privacy-preserving techniques, such as differential privacy, ensured that no sensitive customer information is exposed, even during cross-jurisdictional collaboration.
🟣The result was a more unified, effective approach to combating financial crime on a global scale.
The bottom line? Better results, lower costs. Teams can see operational costs reduced. And proactive detection capabilities enable faster identification of high-risk activity, improving overall risk management.
Time to get ahead with AML
The numbers don’t lie: banks detect less than 1% of the $2 trillion laundered annually. Traditional AML approaches aren’t just falling short—they’re falling behind. While criminals evolve their tactics daily, banks remain trapped in outdated systems and inefficient processes.
Consilient’s Federated Learning solution changes this equation. Our clients are already seeing the results:
✅300% improvement in detection rates
✅75% reduction in false positives
✅Secure collaboration across institutions
✅Full compliance with privacy regulations
It’s time to stop working harder and start working smarter. With Federated Learning, institutions can modernize their AML programs without compromising on privacy or compliance.
Don’t wait for the next wave of financial crime to expose the gaps in your AML program. Contact us today to learn how Consilient can help you catch more criminals while reducing costs.