Rare event detection: how Federated Learning transforms AML
How do you find extremely rare instances of financial crime hidden among millions of legitimate transactions? It’s like finding a needle in a haystack.
Until now. Federated learning changes that. By enabling collaborative model training without centralizing data, it creates a powerful solution to one of banking’s most persistent problems.
When it comes to finding those rare instances of financial crime, federated learning offers new benefits that traditional approaches simply can’t match:
Federated Learning benefits | Why it matters |
Collaboration across silos | Unlocks collective intelligence |
Access to broader target data | Combats data scarcity of rare events |
Better generalization | Avoids local model bias |
Preserves privacy | Critical in sensitive domains |
Local processing possible | Useful in edge-based rare events |
Let me show you why this is a big deal for rare event detection…
Financial crime: the ultimate rare event case
Money laundering is the ultimate rare event case. Criminal transactions are specifically designed to look normal, hiding within millions of daily transactions. Put simply, traditional detection methods struggle with this extreme imbalance.
You know what’s equally frustrating? Firstly, organizations are not innovating. There is a hesitance to even try new technology, the effort is to make existing approaches slightly better. Secondly, banks operate in isolation. Each institution only sees its own small piece of suspicious behavior. Criminals exploit this fragmentation by distributing their activities across multiple institutions, knowing no single bank can see the complete pattern.
> Related: For a detailed look at these challenges and solutions, our article ‘Banking on Collaboration: How to Overcome Institutional Barriers‘ provides valuable insights for organizations beginning this journey. |
Of course, financial institutions try to compensate. They hire thousands of analysts to manually review suspicious activities. They implement rules-based systems that flag unusual transactions. More recently, some have deployed machine learning models trained on their internal data.
But here’s the catch: these isolated efforts produce high false positive rates. Analysts waste time investigating legitimate transactions while missing actual criminal activity. The costs and risks are staggering.
This is exactly why federated learning offers such compelling advantages for financial crime detection. Rather than each bank struggling alone, what if they could collaborate on detection without compromising privacy?
Learning from visual recognition puzzles
With machine learning, there’s a famous challenge that perfectly illustrates the difficulty of rare event detection: distinguishing Chihuahuas from blueberry muffins.
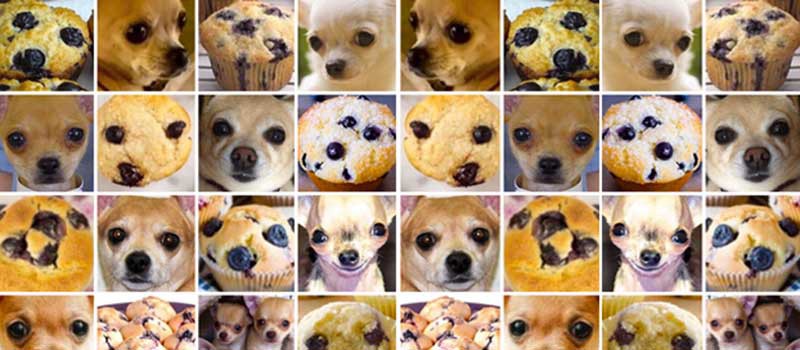
When shown closely cropped images of brown Chihuahuas and blueberry muffins, both humans and AI systems often struggle to tell them apart. The visual similarities (the brown color, texture, and general shape) create genuine confusion despite these being fundamentally different objects.
This visual puzzle parallels the challenge facing AML compliance teams. Money laundering transactions don’t announce themselves with obvious red flags. They’re deliberately designed to blend in with legitimate financial activity. Like finding a single Chihuahua among thousands of muffins, detecting financial crime requires identifying subtle distinctions that aren’t immediately obvious.
The challenge becomes even more daunting when we consider the extreme data imbalance. In a typical financial dataset, legitimate transactions outnumber suspicious ones by thousands or millions to one. Traditional detection systems struggle with this imbalance, either missing the rare events or generating overwhelming false positives.
The patterns that matter in financial crime detection are often invisible to traditional systems. Money launderers distribute activities across multiple institutions, accounts, transactions, and time periods specifically to avoid detection. No single transaction looks suspicious in isolation. Instead, it’s the pattern across transactions that reveals the criminal intent.
This is precisely why machine learning approaches that can identify subtle patterns across vast datasets offer such promise. But there’s a critical challenge: no single institution has enough examples of suspicious activity to train truly effective models on their own.
The solution? A collaborative approach that preserves privacy while enabling collective learning.
The collective intelligence advantage
Single institutions face clear limitations when trying to detect financial crime. Each bank only sees a fragment of the overall picture.
Traditional machine learning models can only learn from the data available within a single organization. With financial crime being so rare, these models have too few examples to effectively identify suspicious patterns.
This is where federated learning comes in and transforms detection capabilities. Rather than centralizing sensitive data, to create enough cases to identify the subtlety of different pictures, federated learning allows models to be trained across multiple organizations while keeping the data exactly where it belongs – inside each institution’s secure environment.
Here’s how it works: each bank trains models on their own data, then shares only the model updates – not the underlying customer information. These updates are aggregated into a stronger collective model that benefits from the combined insights of all participating institutions.
The impact is huge. Consilient’s federated learning approach has proven to be 3x more efficient than traditional methods. Even more impressive, it detects 10% more suspicious cases than single-institution models.
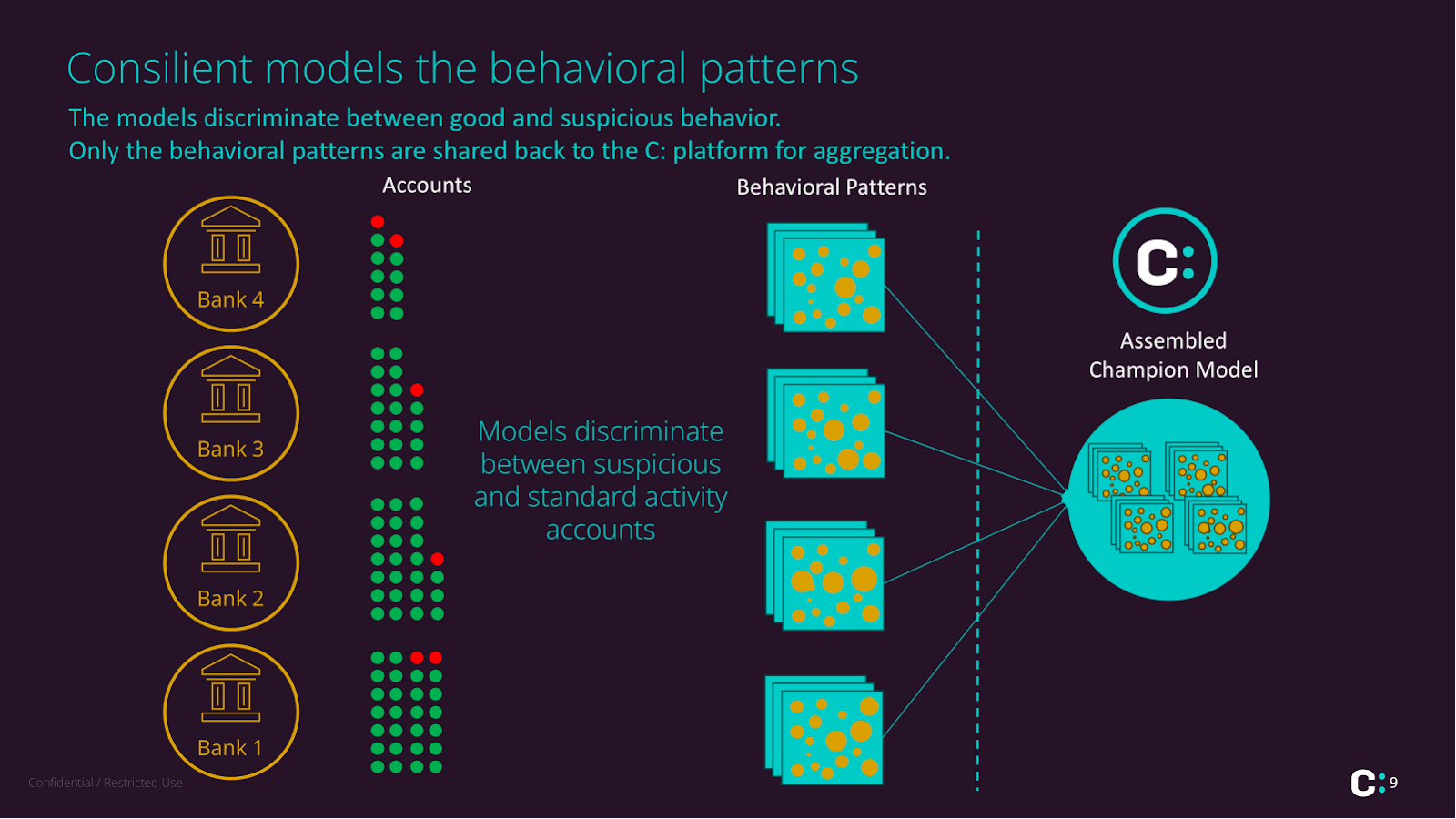
By learning collectively without compromising data privacy, banks can identify patterns that would remain invisible to any single institution. For the first time, financial institutions can recognize coordinated criminal activity that deliberately spans multiple banks.
Naturally, this collaborative advantage extends beyond just finding more cases. It also dramatically reduces false positives, allowing compliance teams to focus their expertise elsewhere.
Why Federated Learning excels at rare event detection
Financial crimes are the perfect example of rare event detection. The scarcity of positive examples makes these cases notoriously difficult for traditional machine learning approaches. Federated learning offers specific advantages that make it uniquely suited to this challenge.
#1. Data scarcity, spread across institutions
The data scarcity problem is fundamental to rare event detection. Money laundering transactions may represent less than 1% of all banking activity. No single institution has enough examples to train truly effective models.
Federated learning solves this by enabling multiple organizations to collaboratively train shared models without exposing sensitive data. The result is access to a much richer, more representative dataset.
#2. Improved generalization
Individual banks develop biases based on their customer demographics, geographic focus, and business model. These biases become more pronounced with rare events due to limited positive examples.
Federated learning creates models that generalize better by incorporating diverse perspectives from multiple institutions.
#3. Privacy preservation is critical
Privacy preservation remains non-negotiable in financial services. Banks must maintain strict compliance with regulations like GDPR while effectively fighting financial crime.
Federated learning addresses this by keeping all raw data local while sharing only model updates. This maintains regulatory compliance while enabling unprecedented collaboration.
#4. Handling class imbalance better
The extreme class imbalance in financial crime detection creates additional challenges. When suspicious transactions represent just one in thousands or millions, models struggle to identify meaningful patterns.
Federated approaches can share knowledge about rare patterns without sharing the underlying data, effectively amplifying the signal from these rare classes.
#5. Real-time and edge use cases
In banking networks, suspicious activities may appear across different parts of the system. Traditional approaches require centralized data collection, creating delays and privacy concerns.
Federated learning enables smart anomaly detection directly where the data originates, learning from distributed patterns without requiring centralized storage. This allows for faster detection of emerging threats.
Looking forward: The expanding potential
The bottom line: Federated learning creates new possibilities for financial crime prevention across institutional boundaries without compromising privacy.
Consilient’s specialized AML models demonstrate how federated learning can target specific financial crime patterns with unprecedented precision.
For financial institutions, the choice is straightforward: continue with isolated systems generating overwhelming false positives, or use collaborative intelligence to improve detection while reducing costs. Ready to transform your approach to financial crime detection? Contact Consilient today to discover how federated learning can enhance your AML effectiveness.