Five high-risk typologies financial institutions frequently miss
Your customer database likely contains several high-risk businesses you don’t know about. These entities didn’t declare their true activities during onboarding—and your AML systems aren’t detecting their actual behaviors.
They’re operating in plain sight, posing serious compliance risks.
The reality is, high-risk typology behavior often goes unnoticed due to a mix of siloed systems, incomplete oversight, and underutilized technology. Even well-resourced compliance teams can miss red flags when customer data, transaction history, and risk intelligence aren’t connected or kept up to date.
And the issue isn’t a lack of data—it’s a failure to interpret the behavioral signals that matter. Transaction patterns speak louder than declarations, yet most financial institutions lack the tools to read that behavioral language.
In this post, we’ll look at the five typologies most commonly missed. But first, we’ll go into more detail on the challenges we’ve just introduced.
Why these typologies are so easy to miss
We know the challenge isn’t a lack of data. It’s the inability to connect it or act on what it reveals.
In most institutions, customer data, transaction activity, and KYC/AML intelligence live in separate systems. This lack of integration makes it difficult to build a complete picture of how a customer behaves across accounts, products, or geographies.
Risk ratings assigned at onboarding are rarely updated. This static, “set and forget” approach means that even when a customer’s behavior changes—such as shifting to international transfers or engaging in high-risk sectors—they may still be treated as low-risk.
Rules-based transaction monitoring systems, meanwhile, rely on rigid thresholds like “flag cash deposits over $10,000.” These rules are:
- Easy to evade (e.g., through smurfing)
- Too broad or narrow to flag nuanced behavior
- Not tailored to specific typologies like trade-based money laundering or human trafficking.
At the same time, typologies evolve—but monitoring systems often don’t. Many financial institutions still don’t use artificial intelligence or machine learning to detect non-obvious or emerging behavior patterns. That leaves them blind to the more complex laundering techniques in play today.
And while technology plays a role, human factors can’t be ignored. Investigators face alert fatigue. Training may be inconsistent and due diligence processes are incomplete. All of this contributes to high-risk clients being onboarded as low-risk… And remaining undetected for far too long.
Here’s where things typically break down:
Cause | Consequence |
Siloed data | No end-to-end customer view |
Static risk ratings | Misses real-time changes in customer behavior |
Poorly tuned monitoring rules | Fails to detect typology-specific activity |
Weak use of AI or behavioral logic | Complex laundering schemes go unnoticed |
Human fatigue or lack of training | Important alerts are ignored or downplayed |
Incomplete due diligence | High-risk clients onboarded as low-risk |
Understanding these gaps is the first step. Next, we’ll break down five high-risk typologies that most frequently slip through the cracks—and cover the specific transaction behaviors that can help you spot them sooner.
1. The hidden Money Service Business (MSB)
MSBs are one of the most challenging high-risk typologies for financial institutions to identify. Why? Because what they covertly offer—money transfers, currency exchange, and check cashing—are all activities that can easily mask money laundering operations.
Why they’re missed:
The typical hidden MSB doesn’t register as such. Instead, they operate under retail or service business fronts. A convenience store, mobile phone shop, or small grocery might process dozens of money transfers daily without disclosing this activity to their bank.
Behavioral indicators:
🚩 High volumes of cash deposits followed by wire transfers to high-risk jurisdictions
🚩 Unusually high transaction velocity compared to the stated business type
🚩 Frequent transactions just below reporting thresholds
🚩 Inconsistent business revenue patterns compared to similar businesses
Detection challenges:
Traditional KYC, CDD, and EDD processes often rely heavily on customer-provided information. Once a business is categorized, many financial institutions lack the behavioral analytics needed to detect when actual transaction patterns deviate from the stated business purpose. This makes it difficult to identify hidden money laundering activities that are masked by seemingly legitimate operations.
2. Not-for-Profit Organizations (NPOs) (operating outside disclosed activities)
NPOs serve vital social purposes, but they’ve also become vehicles for financial crime when operating outside their declared mission.
Why they’re missed:
Financial institutions may apply lighter scrutiny to NPOs due to their charitable status and public trust. This can create vulnerabilities, allowing some organizations to engage in financial activities that are inconsistent with their stated objectives, potentially facilitating money laundering or terrorist financing..
FATF emphasizes that not all NPOs are high-risk, and urges governments and FIs to apply a risk-based approach, not blanket restrictions. Therefore leading to this type of organization going unchecked.
Behavioral indicators:
🚩 Funds moving to regions unrelated to the NPO’s declared mission
🚩 Transaction patterns inconsistent with typical donation cycles
🚩 High-value transactions with commercial entities that don’t align with nonprofit activities
🚩 Unusual cash withdrawal patterns
Detection challenges:
Banks typically assess NPO risk based on their mission statement and registration documents. However, transaction monitoring systems rarely flag behavior that contradicts these documents unless specific rules are created—which most institutions lack.
3. Covert Virtual Asset Service Providers (VASPs)
As cryptocurrency adoption grows, so does the number of businesses facilitating crypto transactions. The problem is, many operate without proper registration or disclosure to their financial institutions.
Why they’re missed:
Undisclosed VASPs typically present themselves as technology companies, consultancies, or online services. Traditional AML systems weren’t designed to identify the transaction patterns associated with crypto facilitation.
Behavioral indicators:
🚩 High volumes of transactions with known cryptocurrency exchanges
🚩 Frequent, round-number transactions with multiple counterparties
🚩 Unusual patterns of international wire transfers
🚩 Inconsistent business revenue compared to stated business model
Detection challenges:
Most financial institutions use static rules to monitor transactions. These systems can’t identify the complex patterns that indicate a business is secretly operating as a VASP. Additionally, many banks lack reference data about cryptocurrency exchanges to properly flag these connections.
4. Undeclared precious metals dealers
Precious metals remain an attractive vehicle for money laundering due to their high value, portability, and relatively stable prices.
Why they’re missed:
Businesses dealing in precious metals often present themselves as jewelry retailers, import/export companies, or general trading businesses. Without specialized detection capabilities, banks struggle to identify when these businesses are primarily engaged in high-volume precious metals trading.
Behavioral indicators:
🚩 Frequent transactions with known refineries or metals wholesalers
🚩 Unusual patterns of international transactions with gold-producing regions
🚩 Cash-intensive operations inconsistent with typical retail jewelry sales
🚩 High transaction values compared to stated business size
Detection challenges:
Traditional transaction monitoring relies heavily on transaction amount and frequency. It lacks the ability to analyze counterparty relationships and transaction contexts that would reveal undisclosed precious metals trading.
5. Casino-like operations (under commercial fronts)
Underground gambling operations are significant money laundering risks, yet they frequently operate through seemingly legitimate businesses.
Why they’re missed:
These operations typically use entertainment venues, clubs, or retail businesses as fronts. Their banking activities appear legitimate without advanced behavioral analysis.
Behavioral indicators:
🚩 High-volume cash deposits during unusual hours
🚩 Frequent transactions with known gambling-related entities
🚩 Inconsistent revenue patterns with sudden spikes
🚩 Unusual velocity of funds moving through accounts
Detection challenges:
Most transaction monitoring systems focus on individual transactions rather than behavior patterns over time. This makes it difficult to identify the consistent patterns that would expose covert casino operations.
Consilient’s High-Risk Typology Model uses 15+ specialized typology scores to identify these behaviors—learn more about our approach. |
The cost of missing these typologies
The financial and regulatory impact of failing to identify hidden high-risk typologies goes beyond the immediate penalties. These costs cascade through multiple areas of your organization:
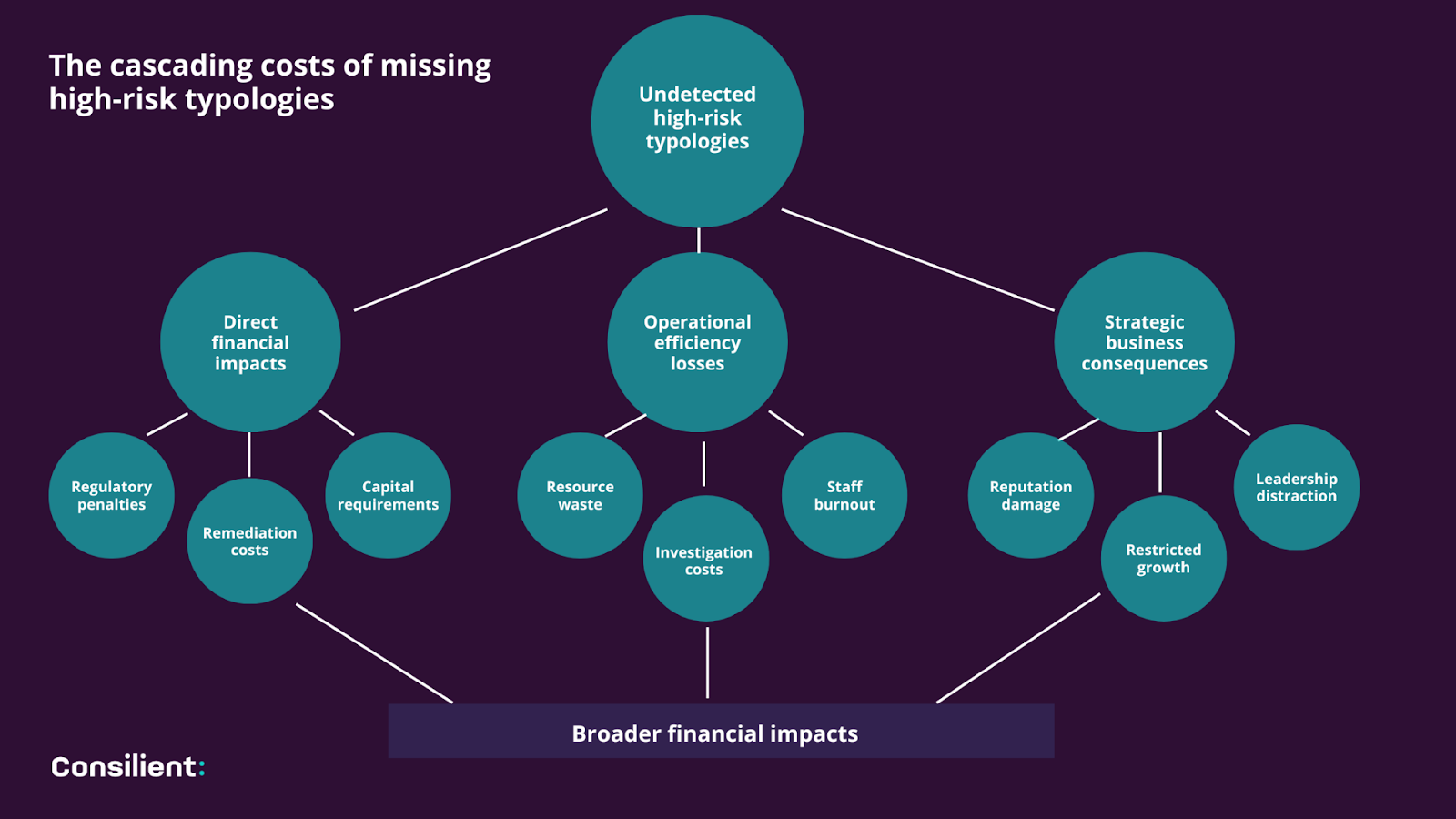
Direct financial impacts
⏹️ Regulatory penalties continue to escalate. Financial institutions now face penalties in the hundreds of millions for AML failures. The trend shows regulators increasingly focusing on typology detection failures specifically. Several recent enforcement actions have explicitly cited inadequate typology risk assessment.
⏹️ Remediation costs often exceed the penalties. When regulators identify control weaknesses, the cost of mandated lookback exercises, external consultants, and system upgrades can reach 2-3 times the initial fine amount. Industry analysts consistently report that total remediation costs can reach several multiples of the original penalty amount.
⏹️ Capital requirements increase with compliance failures. Regulators frequently impose higher capital adequacy requirements on institutions with demonstrated AML weaknesses, tying up funds that could otherwise generate returns.
Operational efficiency losses
⏹️ Compliance teams waste valuable resources investigating false positives while missing genuine risks. Industry data shows that up to 95% of alerts generated by traditional systems are false positives (according to a 2020 report by Global Investigations Review). Meanwhile, hidden high-risk typologies continue operating undetected.
⏹️ Investigation costs skyrocket when typologies are discovered late. When high-risk activities are only identified after substantial transaction history has accumulated, the required lookback investigations demand significantly more resources than proactive identification.
⏹️ Staff burnout increases with ineffective systems. Compliance professionals face growing frustration when their time is spent on unproductive investigations rather than addressing genuine risks, leading to higher turnover in critical compliance roles.
Strategic business consequences
⏹️ Reputational damage causes lasting harm. Financial institutions associated with financial crime face measurable impacts on customer acquisition, business partner relationships, and investor confidence. These effects typically persist for 18-24 months following public enforcement.
⏹️ The opportunity cost of restricted growth is substantial. Institutions under regulatory scrutiny often face limitations on expansion, new product development, and market entry until remediation is completed.
⏹️ Board and executive focus diverts from strategic priorities. When serious compliance failures emerge, leadership attention necessarily shifts from growth initiatives to crisis management.
Broader financial system impacts
⏹️ Financial crime proliferation continues unchecked. Ineffective detection enables criminal networks to expand operations using legitimate banking systems, creating even larger future challenges.
⏹️ Regulatory expectations continue rising. Each major compliance failure leads to heightened standards and expectations for all institutions, creating an escalating compliance burden across the sector.
⏹️ Trust in financial institutions erodes with each publicized failure, making regulatory compliance not just a legal requirement but an essential component of maintaining public confidence in the financial system.
See how our federated learning technology identifies behavioral patterns that traditional systems miss. |
Beyond rules-based detection
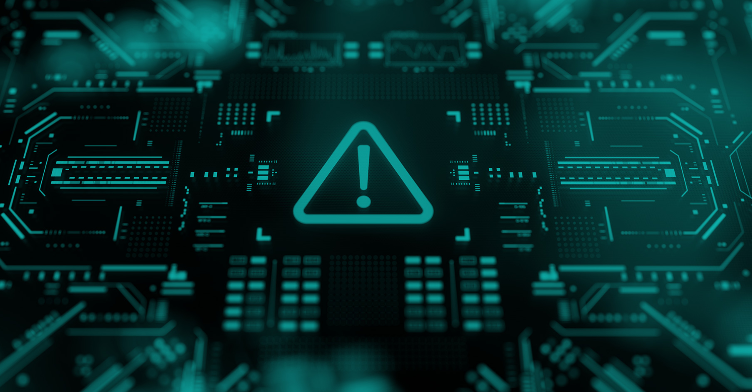
The persistence of these hidden typologies demonstrates the limitations of conventional AML approaches. Rules-based systems can only identify known patterns, while criminals constantly evolve their methods.
Effective detection requires a fundamental shift from static rules to behavioral analysis. This means:
- Analyzing transaction patterns over time rather than focusing on individual transactions.
- Understanding the detailed behavior of these types of companies compared to non-high risk company typologies.
- Leveraging data from multiple sources to create a comprehensive view of customer behavior.
- Using advanced analytics to identify subtle patterns human analysts might miss.
- Implementing continuous learning systems that adapt to evolving criminal tactics.
Financial institutions that successfully detect these hidden high-risk typologies share one common approach: they focus on behavior, not declarations. They implement systems capable of identifying when a customer’s actual transactions don’t match their stated business purpose.
📖For a deeper dive into how Consilient’s model addresses these challenges, read our latest blog: How Consilient’s new model detects hidden high-risk typologies. |
A new approach to typology detection
Detecting these hidden high-risk typologies requires looking beyond what customers tell you to what their transactions reveal. Modern approaches using behavioral analysis and machine learning can identify businesses operating in high-risk sectors even when they haven’t disclosed their true nature.
The most effective high-risk typology detection systems can:
✅ Analyze 12+ months of transaction behavior to establish accurate customer profiles
✅ Identify specific patterns associated with high-risk typologies
✅ Compare customer behavior to known patterns of similar businesses
✅ Adapt to new and emerging financial crime methods
Financial institutions need solutions that can close the gap between what customers declare and how they actually behave. This requires moving beyond traditional rules-based monitoring to systems capable of identifying subtle behavioral patterns.
Identifying and targeting high-risk company types is a challenging task. Companies can only accurately assess risk if they have enough behavioral patterns to draw from, but these patterns are often rare. This scarcity makes it difficult to develop robust models for identification. Federated machine learning offers a solution by enabling organizations to share the characteristics of these patterns without disclosing actual data. This collaborative approach creates a unique opportunity for companies to leverage insights across the industry in order to better identify high-risk typologies.
Take action now
The cost of missing hidden high-risk typologies grows each year. Financial institutions must act now to enhance their detection capabilities.
Start by assessing how effectively your current systems can identify undisclosed high-risk typologies. Ask yourself:
- Could your systems detect an MSB operating under a retail front?
- Would you identify an NPO sending funds to regions unrelated to its stated mission?
- Can you recognize the transaction patterns of an undisclosed VASP?
The answers to these questions will reveal whether your institution is prepared to meet modern financial crime challenges—or whether criminals are operating unchecked within your customer base. We’d genuinely love to help you strengthen your typology detection capabilities. Contact us today to learn how advanced behavioral analysis and federated learning can transform your ability to identify hidden high-risk customers.