ABA Bank Compliance Magazine: AI and the Future of Bank Compliance: How Do You Know If It Is Working?
This article by Gary M. Shiffman originally appeared the ABA Bank Compliance Magazine.
Artificial Intelligence democratizes; it is the great equalizer that allows non-technical people to perform complex and previously unthinkable tasks using technology, without writing a single line of code. Bullet-proof hosting and pseudonymous identities, for example, enable money laundering, sanctions violations, and frauds against consumers such as romance and old-age scams and identity theft. Artificial Intelligence will also add to the complexity of the products being sold by banks to the public.
Artificial Intelligence and Machine Learning (AI/ML) absolutely complicate a professional life in compliance. But I am writing this article to encourage bank compliance professionals to see AI/ML as the enabler to accomplish fantastic improvements in the compliance profession. AI/ML is not magic, however, and it will endow people with powers only if time is taken to learn how it works, and more importantly, how to know if it is working.
When people are unsure of the facts or the interpretation of the facts, they typically make judgments based on biases. For example: does the person seem competent? Do my peers in the industry use that brand of software? When stated this way, it is obviously the wrong way to measure a program’s performance. But judging a compliance program based upon bias is a recipe for failure. In fact, one of the goals of compliance is to guard against biases, intentional or otherwise. Instead, by measuring results, technology can give confidence in facts and interpretations. The purpose of this article is to address the measurement of AI/ML systems, so compliance professionals can have the power to make sound judgments on the effectiveness of AI/ML systems.
Training Data and Testing Data
The sheer enormity of available data today makes AI/ML possible. Algorithms learn by example; the more data, the better the algorithms become. When we type the word “cat” in an internet search bar, we take for granted that Google presents us with countless images of cats. The Google cat algorithm represents iconic machine learning, specifically a subset called computer vision. Let a five-year-old child play with a cat for 15 minutes, and that young human will learn, through deduction, how to recognize other cats. The cat algorithm, however, required 10,000,000 images of cats before it started to learn – inductively – to distinguish cat images from all the other images on the internet.
Extending this inductive learning process to compliance: to identify money laundering or consumer fraud schemes or biased banking practices using AI/ML, examples of these behaviors must be fed into an algorithm. Data trains the algorithm. A lot of good data trains excellent algorithms.
Scientists often talk about “ground truth” data – data you know is labeled correctly. Pictures of cats properly labeled as a cat is a good example of ground truth data. One requires a lot of ground truth data to train an algorithm. Likewise, ground truth data is necessary to test your now-trained algorithm. Your test data serves as your answer key. Like a teacher testing a student, you give data to an algorithm and test the ability of the technology to sort the data based on the attributes you value, such as similarity to the likeness of a cat, or similarity to the behavior of a money launderer or fraudster.
In the world of AI/ML, scientists often talk about the output as a “prediction,” but this is not used in the sense of seeing into the future; instead, think of classification. The testing of an algorithm is the testing of the ability of the algorithm to classify the source of the data. Of all the data that is run through the algorithm, what is the probability that the next image is a cat? Or a money launderer? Or a consumer fraudster?
DATA → ALGORITHM → DISTRIBUTION → THRESHOLD → MEASURE
Humans need to test these algorithms to find out how well they perform. It is important to note that the actual output of a ML algorithm is a distribution; a human must then actually draw the threshold. Everything above a threshold would be deemed as predicted “true,” or requiring human review. Everything below that threshold would be predicted “false.” So testing the performance of an AI/ML system requires measurement of a technology-generated distribution at a human-drawn threshold.
Before I proceed, please notice the indispensable role of the human. AI/ML, properly conceived and deployed, empowers humans and does not replace humans.
To make it easy, I will start with a popular internet meme: chihuahuas and muffins.
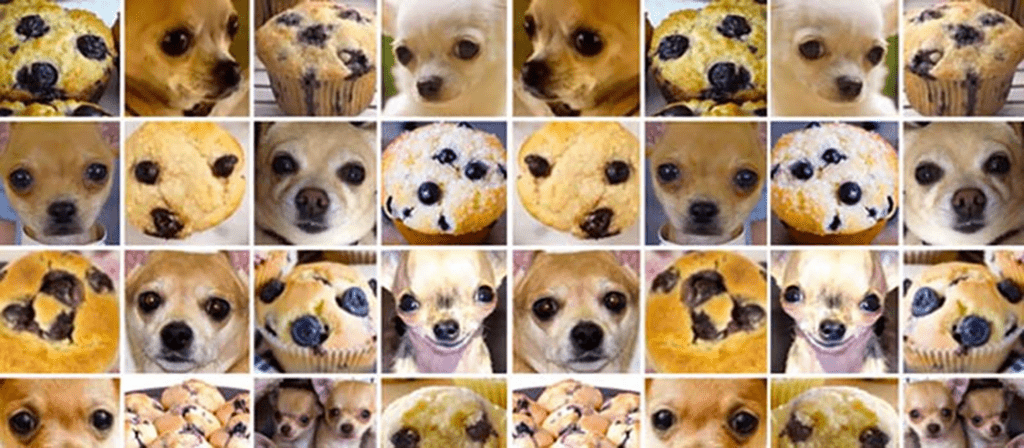
[Karen Zach, March 9, 2016. https://twitter.com/teenybiscuit/status/707727863571582978]
Building on the cat image description of computer vision above, I want to measure an algorithm’s ability to distinguish chihuahua from not-chihuahua, and to make it difficult, I will use images of blueberry muffins to confuse the algorithm.
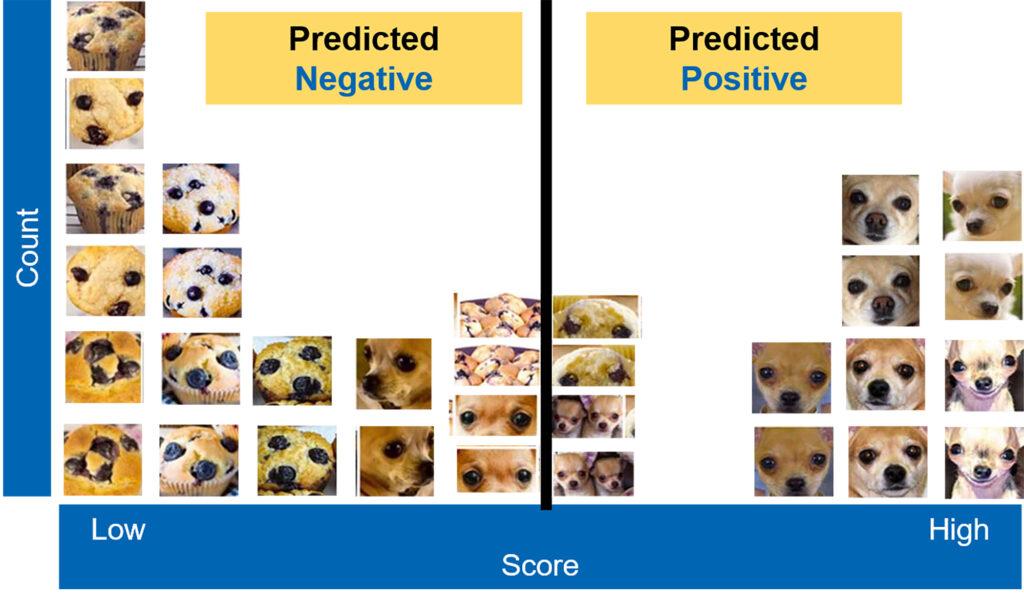
With this data, the images mixed together, we run the data through an algorithm, the output of which is a distribution.
Measurement implies the use of numbers – math. After two decades teaching at Georgetown University, I understand that many people feel a bit of anxiety when math comes up. But to move from bias to empirical measurement, we need numbers. To keep it simple, I am asking that our community count just four items: true positives, false positives, true negatives, and false negatives (sometimes just noted as TP, FP, TN, and FN). Using testing data and counting after drawing a threshold, we can measure ML performance. How well can a machine identify a money launderer or a consumer scam? Count these four items, and you can measure performance.
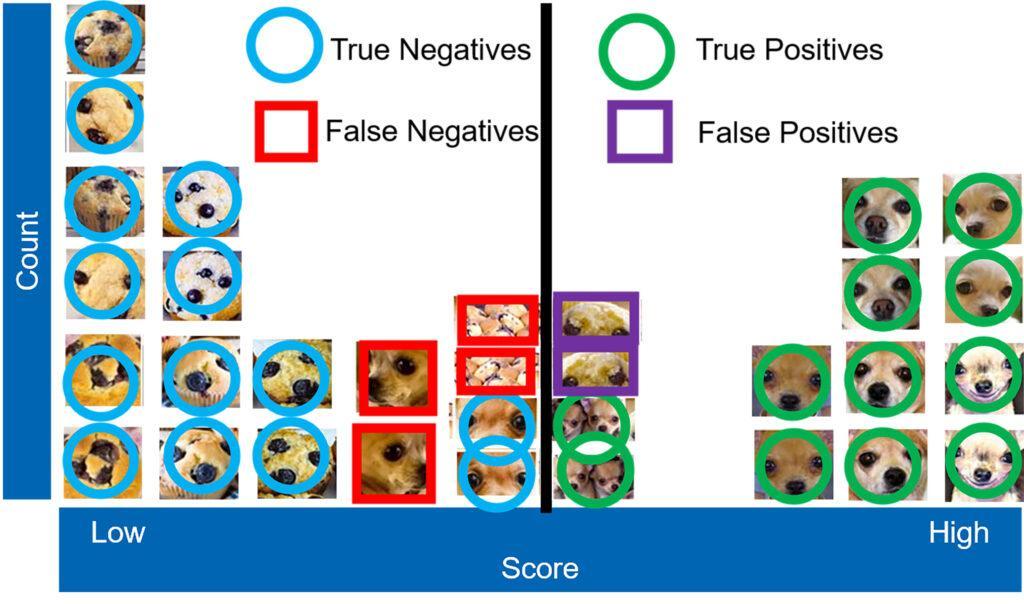
A true positive means the machine identified the image of a chihuahua above the threshold correctly as a chihuahua. A false positive means the machine identified the image of a muffin as a chihuahua — predicted positive, but false: a false positive. A true negative means the machine correctly identified the image as a muffin/not-chihuahua. A false negative means the machine identified the image as a muffin/not-chihuahua, but it was actually a chihuahua and should have been sorted to the right of the distribution line — predicted negative, but falsely: a false negative.
Once counting is done, you may realize that counting does not yet easily tell the story of empirical performance. Counting provides the building blocks for answering the question, “Is it working?” In guidance coming from Congress and the regulators, the terms “efficiency” and “effectiveness” often get used. Thanks to the academic disciplines from which ML comes, these terms can take on empirical meaning by converting the counts of TP, FP, TN, and FN into a comparable measurement.
Precision
Think of “efficiency” as the percentage of accurate predictions in the population identified for human review. Of everything predicted chihuahua, what percent were actually chihuahuas (true positives)? You’ve heard complaints for years – you may have voiced some – about systems generating too many false positives. Maybe you review too many alerts that never become a good case because of errors in name matching, for example. “Precision” measures efficiency, for those who want the math. Precision equals True Positives divided by all Positives.
Precision = _______True Positives_______
(True Positives + False Positives)
From a banker’s perspective, minimizing false positives may be the biggest challenge facing the governance, risk, and compliance functions. You can compare precision of a system over time, or compare precision across competing systems to understand and measure performance in the dimension of efficiency.
Recall
If you are a regulator, or other enforcement official of the government, you may not articulate false positives frustration, but you may instead worry about the state of false negatives in the banking system. A false negative occurs when you miss exactly what you are looking for; you miss a money launderer or a fraudster. In our simple example here, a false negative is a chihuahua below the threshold and therefore predicted as a muffin.
Think of “effectiveness” as the percentage of accurate predictions in the population intended to be reviewed. Of the total chihuahuas in the population, how many were sent for human review? That percentage is called “recall.”
“Recall” measures effectiveness. For those who want the math, recall equals True Positives divided by all True:
Recall = _______True Positives_______
(True Positives + False Negatives)
Recall is a score of the likelihood that a money launderer was identified by the algorithm, for example.
Replacing “chihuahua” with “predator,” we see that the stakes are high, and that the compliance mission is important. Uncertainty in facts and the resulting biases help no one. Resisting the encroachment of AI/ML into the compliance space will prove futile. Measurement of AI/ML systems provides the tools needed to navigate into the future. Get good training data to build algorithms, then regularly test those algorithms. Imagine AI/ML output as a distribution. Then evaluate human judgment in placing the thresholds — what gets reviewed and what gets missed — using the language of effectiveness and efficiency. While Artificial Intelligence and Machine Learning technologies can certainly complicate compliance, measurement will enable this noble mission of compliance and let us know when the systems are working.