How Consilient’s new models detects hidden high-risk typologies
Banks are unknowingly harboring undisclosed high-risk customers—and outdated AML models aren’t catching them.
Financial criminals move billions through MSBs, Casinos, NPOs, Precious Metals Dealers, and VASPs every year. Many operate openly, but some remain hidden within a bank’s existing customer base, bypassing traditional transaction detection models. Without clear visibility, financial institutions risk regulatory scrutiny, compliance failures, and operational inefficiencies, and critically illicit funds flowing through their organization.
For banks, the biggest challenge is uncovering undisclosed high-risk typologies. That’s why Consilient has launched its new High-Risk Typology Models—a federated machine learning-powered solution that detects hidden financial crime without data sharing.
Using 15+ AI-driven high-risk typology models built on 12 months of behavioral data, the solution enables banks to identify customers potentially operating in high-risk sectors, carry out appropriate EDD, and strengthen compliance—all while preserving privacy.
So, how can banks get ahead of hidden risks? Read on to learn how leading institutions are enhancing AML detection with federated learning.
The AML detection gap: Why banks are missing high-risk entities
High-risk sectors are prime targets for financial crime, yet most banks lack the tools to detect and manage them efficiently. Money Service Businesses (MSBs), Casinos, Not-for-Profits (NPOs), Precious Metals Dealers, and Virtual Asset Service Providers (VASPs) pose significant AML risks, but traditional models fail to identify customers operating within these industries, especially when they don’t disclose their true activities, exposing the financial institution to significant risks.
🔴 Hidden high-risk typologies behaviors = hidden threats. Criminals rarely register as MSBs or VASPs. Instead, they operate under the radar, structuring transactions to avoid scrutiny. Without typology-driven behavioral analysis, banks miss key red flags.
🔴 Rules-based systems are always one step behind. Static AML models flag known risks—not emerging threats. Financial crime is continuously evolving, and banks relying on legacy systems are reacting, not preventing.
🔴 Risk Models and Controls Depend on Accurate Classification. If a business behaves like a casino but is treated like a restaurant, the wrong controls get applied. This means inadequate monitoring, low alert thresholds, and missed red flags.
🔴 Behavioral patterns reveal what customer declarations don’t. Consilient’s research has identified 15+ high-risk typologies, each with distinct transaction behaviors. From cash-heavy MSBs to charitable organizations misused for illicit funding, these sectors require behavior-driven detection models—not just static rules.
🔴 Fragmented data creates blind spots. Banks relying on internal transaction data and static watchlists only see part of the picture. Criminals spread activity across institutions, knowing that siloed systems won’t connect the dots.
🔴 Regulators are losing patience. Inadequate typology detection leads to AML compliance failures, fines, and reputational damage. Regulators expect banks to identify risks before they escalate—traditional AML models aren’t cutting it.
High-risk typologies won’t show up on a watchlist—they must be identified through patterns and behaviors. That’s where Consilient’s High-Risk Typology Models come in.
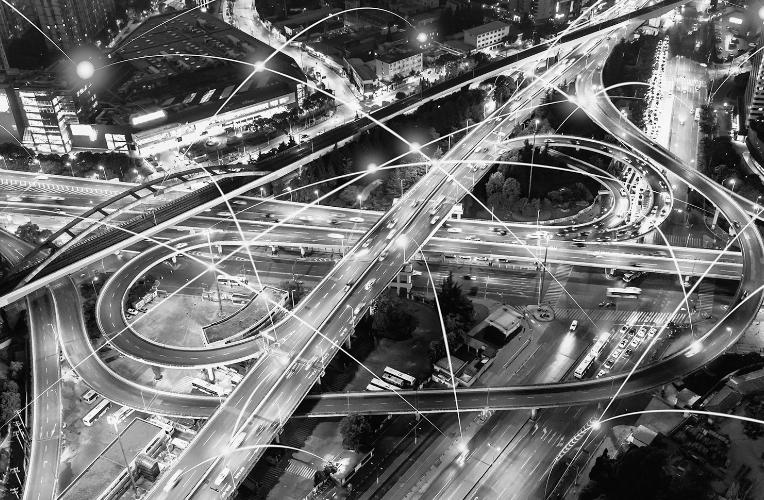
How Consilient’s high-risk typology model solves these challenges
Of course, hidden high-risk typologies won’t expose themselves. That’s why banks need the right tools to uncover them. That’s exactly what Consilient’s high-risk typology model delivers.
Using 15+ AI-driven high-risk typology scores, built on 12 months of behavioral data of the activity of these typologies, the model identifies undisclosed high-risk customers, detects emerging threats, and strengthens risk management at scale.
#1. 15+ high-risk typology scores for unmatched detection
Designed to analyze and identify customer behaviors across MSBs, Casinos, NPOs, Precious Metals Dealers, Arms and Gun shops, Marijuana dealers, and VASPs—even when customers haven’t disclosed their true activities.
Traditional systems depend on static classifications, missing businesses that operate covertly within high-risk sectors. By assessing behavioral patterns, rather than just customer labels, banks gain real-time risk insights beyond what static rules can deliver.
#2. Multi-institution collaboration without data sharing
Most banks rely only on their own internal transaction data, creating blind spots that criminals exploit. Some organizations have strict criteria on the type of companies they will not deal with because of the risk, and therefore, if they are unknowingly dealing with these organizations they do not have the tools to identify them.
Federated learning changes that. Instead of sharing raw data, banks collaborate securely, leveraging collective intelligence of high-risk typology transaction patterns without breaching privacy regulations. This strengthens risk detection across institutions while maintaining full data security and compliance.
#3. Continuous learning to outpace criminal tactics
Money laundering tactics constantly evolve, yet most AML systems struggle to adapt—leaving banks reacting instead of preventing.
Consilient’s model continuously learns and evolves, detecting adversarial money laundering tactics and shifting payment behaviors. As criminals adjust their strategies, the model updates in real-time—so banks stay ahead.
#4. XGBoost-powered Machine Learning for accuracy and scale
Traditional monitoring tools suffer from inefficiencies—they’re slow, overloaded with false positives, and struggle to scale.
Built using XGBoost (Extreme Gradient Boosting), Consilient’s model delivers:
✔️ Fast, highly accurate detection for high-volume transactions.
✔️ Feature importance and overfitting control, reducing false positives.
✔️ A bias-resistant approach to AML risk modelling, validated in real-world banking environments.
#5. Privacy-first AI with local precision and global insights
Banks need a bigger-picture approach to financial crime, but data privacy regulations make information-sharing difficult.
Consilient’s Ensembling technique solves this. By combining local risk signals with global financial crime intelligence, banks gain broader insights into high-risk typologies—without centralized data pooling.
A smarter, more effective way to detect high-risk customers
Instead of drowning in false positives and missing hidden risks, banks using Consilient’s High-Risk Typology Model can:
✔️ Detect undisclosed high-risk customers before regulators do.
✔️ Strengthen AML defenses while reducing operational strain.
✔️ Enhance typology detection with AI-driven insights—without sharing sensitive data.
High-risk typologies won’t expose themselves. Banks need the right tools to uncover them. That’s exactly what this model delivers.
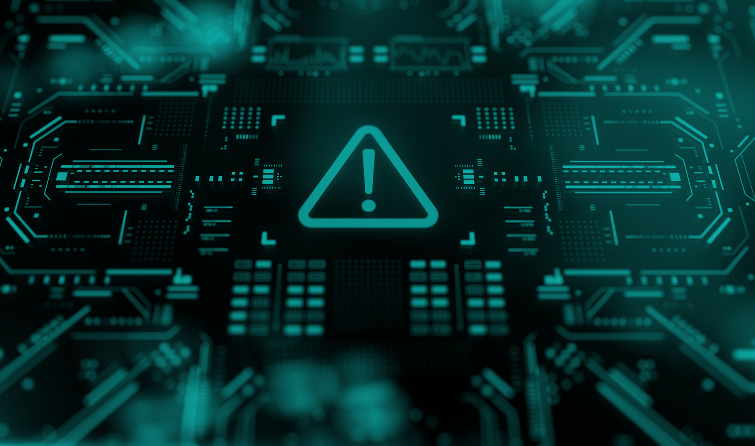
The business case: Why banks need this model now
Regulators are raising expectations, compliance costs are rising, and financial criminals are becoming more sophisticated. Banks can’t afford to rely on outdated AML models that miss hidden high-risk customers and drown teams in false positives.
Consilient’s High-Risk Typology Model changes that. Designed for modern financial crime challenges, it proactively detects risk, enhances compliance, and improves operational efficiency—without disrupting existing systems.
🔹 Proactive Customer Due Diligence (CDD) for risk detection
Banks need to identify high-risk customers before they become liabilities. Under FATF Recommendation 10, organizations must understand their business purpose and monitor activity. Ensuring that the actual behavior is aligned with the known business purpose is vital. Using Consilient, organizations can detect high-risk behaviours that were not disclosed and align to high-risk typologies.
✔ Detects MSBs, Casinos, VASPs, NPOs, and other high-risk businesses—even when customers fail to declare their true activities.
✔ Reduces financial losses, regulatory penalties, and reputational damage by ensuring hidden risks don’t go unnoticed.
🔹 Enhanced Due Diligence (EDD) risk insight
FATF and National regulators expect financial institutions to detect and manage high-risk typologies—not just react after unusual activity. Ensuring that organizations can monitor and check entities that appear to operate within a high-risk sector and with unusual transactions is key. Consilient’s models provide typology risk scores to identify entities that may be operating in these sectors.
✔ Aligns with evolving AML/CTF requirements using advanced typology detection.
✔ Ensures compliance with global standards while improving the detection of unseen and unknown risks.
🔹 Operational efficiency gains
High false positives waste resources, slow investigations, and prevent compliance teams from focusing on real threats.
✔ Automates high-risk typology detection, reducing reliance on manual reviews and rule-based systems.
✔ Optimizes compliance workflows, freeing up resources for high-value investigations and remediation.
🔹 Compliance leadership
AML innovation means staying ahead of financial crime, strengthening risk detection, advancing regulatory compliance, and setting the standard for compliance excellence.
✔ Strengthens institutional resilience with an adaptive, real-time AML solution.
✔ Positions financial institutions as AML leaders, leveraging federated learning for stronger risk detection while maintaining data privacy.
Now is the time to move beyond outdated, rules-based AML models and adopt a proactive, privacy-first approach to financial crime detection.
Uncover hidden high-risk sectors, ensure compliance, preserve trust
Traditional AML systems weren’t built for today’s financial criminals and their ability to move illicit gains. Hidden high-risk customers slip through due diligence, compliance teams are buried in false positives, and institutions face mounting regulatory pressure.
It’s time for a smarter, more effective approach.
Consilient’s High-Risk Typology Model delivers next-generation AML detection, allowing banks to identify undisclosed high-risk activity, enhance compliance, and optimize operations without compromising privacy.
With:
✔️ Advanced high-risk typology detection built on 12 months of behavioral data.
✔️ Federated learning-powered collaboration to close financial crime blind spots.
✔️ Continuous learning to stay ahead of evolving criminal tactics.
Financial institutions can now strengthen AML strategies, reduce false positives, and safeguard financial integrity—all without disrupting existing systems. Why wait? Criminals aren’t slowing down, and regulators aren’t lowering their expectations. Take the next step today: Talk to us—we’d genuinely love to help.