Anomaly detection at scale: Consilient’s approach to high-risk entities
Financial crime has never been more sophisticated. Bad actors constantly evolve their tactics, exploiting gaps between systems and the sheer complexity of global finance. Banks detect less than 1% of the $2 trillion laundered annually—a reminder that traditional AML tools aren’t keeping pace.
The problem? Most banks still rely on isolated data and rigid rules that can’t adapt to new threats. High false positive rates waste resources, while truly suspicious patterns remain hidden. Banks are fighting modern criminals with outdated weapons.
Consilient tackles this challenge through Federated Learning—a privacy-preserving approach to collaborative AI. By helping institutions detect anomalies at scale while protecting sensitive data, we’re closing the gap between criminals and the tools designed to catch them. Keen to learn more? Take a look at the post below…
Why banks miss 99% of financial crime
Banks invest heavily in AML technology, yet criminals keep slipping through. Here’s why traditional approaches aren’t working.
Limited view of criminal activity 🔍
Most banks work in isolation, spotting suspicious activity using only their own data. Criminals know this—they deliberately spread their activities across multiple institutions, knowing that no single bank can see the full picture.
Over-reliance on rules-based systems 🔁
Traditional systems rely on preset rules to flag suspicious behavior. While these rules catch known patterns, they can’t adapt to new threats. Criminals quickly learn and exploit these blind spots, leaving banks constantly playing catch-up.
The false positives problem ❌
Up to 99% of system alerts are false positives. This means teams waste countless hours investigating legitimate transactions while real threats slip by unnoticed. This inefficiency diverts resources, inflates costs, and delays the identification of genuine high-risk activity.
Growing regulatory pressure 🔝
Banks face mounting pressure to meet complex AML and counter-terrorism financing (CFT) requirements. These requirements strain already overstretched teams, amplifying the inefficiencies inherent in traditional detection methods.
The bottom line? Without addressing these core weaknesses, institutions will continue to struggle to keep pace with the growing sophistication of financial crime.
Where Consilient’s federated learning steps in
Consilient takes a fundamentally different approach to catching financial criminals. Consilient overcomes the limitations of traditional AML systems, enabling institutions to collaborate effectively without compromising data privacy.
Unlike traditional systems that rely on isolated datasets or centralize data for analysis, Federated Learning shares insights—not data. Models are trained on local data at each institution, learning from unique patterns of behavior within their specific environments.
These insights are then aggregated to build smarter, more adaptive models that capture broader patterns of financial crime across institutions. This means institutions get market-wide intelligence without exposing customer information.
How it works
Federated learning follows a simple but powerful process:
Step 1: Start with a common model
We send the same baseline model to all participating banks. Think of it as giving everyone the same set of initial detection rules.
Step 2: Learn from local data
Each bank trains this model on their own data, teaching it their unique patterns of customer behavior and risk. The model never leaves the bank’s secure environment.
Step 3: Share only the learnings
Banks send back only the encrypted model updates—mathematical insights that contain no customer information. It’s like sharing the rules the model learned without sharing any examples.
Step 4: Build a smarter global model
Our platform combines these insights from all participating banks to create a “champion model.” This model understands patterns of financial crime across the entire network while keeping each bank’s data private.
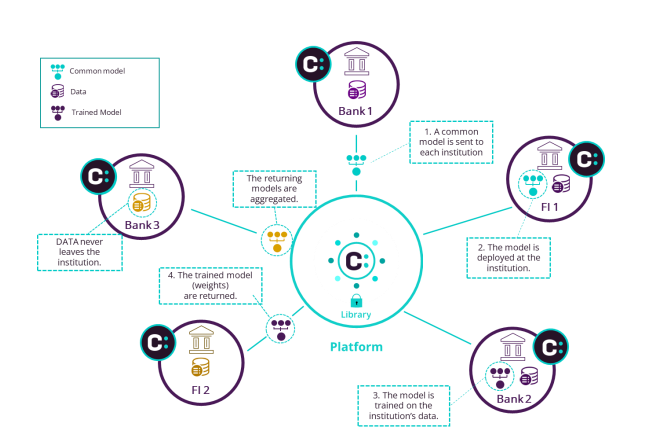
The result? A continuously learning system that gets smarter with every transaction while maintaining the highest standards of data privacy.
Privacy at the core
No sensitive data ever leaves your bank—period. By sharing only encrypted model updates, banks can collaborate while meeting the strictest privacy requirements. This builds trust and keeps customer data secure while fighting financial crime more effectively.
This approach makes risk detection smarter, more efficient, and more secure. It’s exactly what banks need to stay ahead of sophisticated criminals.
Tackling anomalies at scale
The ability to detect financial crime is only as effective as the data and models that power it. Traditional systems, while useful, are limited by the isolated datasets they operate on, which often fail to capture the full spectrum of suspicious activity.
This is where Consilient’s Federated Learning solution steps in. It allows banks to see the complete picture.
Finding hidden patterns
When banks share insights through Federated Learning, they uncover risk patterns invisible to individual institutions. By aggregating insights across organizations, the model becomes more robust and adaptable, capable of detecting anomalies that would otherwise go unnoticed. This means banks can spot emerging threats from global or systemic trends, even when these patterns aren’t visible in their local data.
Key types of anomalies detected
Consilient is particularly effective at detecting complex financial crime that traditional tools miss. Some of the key anomalies that can be detected include:
🟣Unusual transaction behaviors linked to new payment channels: With the rise of new payment systems and digital currencies, traditional tools struggle to keep up with novel transaction patterns. Consilient’s model can identify unusual behaviours tied to these evolving channels, which might otherwise fly under the radar of older detection systems.
🟣Sophisticated activity missed due to isolated data: Criminals deliberately spread their activities across banks, knowing no single institution will spot the full pattern. Many financial crimes are nearly impossible to detect when data stays locked in institutional silos. Consilient, by aggregating insights from a wide array of institutions, ensures that these sophisticated activities are flagged, even when they might appear innocent in any one dataset.
But don’t take our word for it, here are some real results.
Proven results: Efficiency and cost savings
A leading U.S. bank put our solution to the test. Using Federated Learning, they achieved 4x better anomaly detection than their traditional system. Not only did they catch risks they’d never seen before, but they also significantly reduced their operational burden and false positives. In fact, Consilient improved efficiency by 75%.
The overall result? Faster detection, better compliance, and less strain on AML teams.
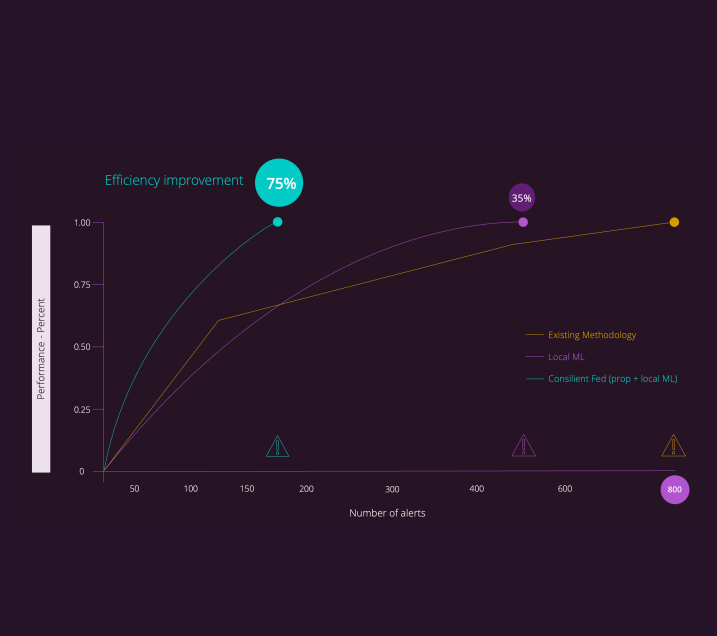
This is what next-generation risk detection looks like: smarter, more efficient, and able to catch criminals no matter how they try to hide. While traditional systems fall behind evolving threats, Consilient’s federated approach helps institutions stay ahead.
Why leading banks work with Consilient for anomaly detection
Traditional systems generate 99% false positives—we significantly lower this rate, letting banks focus resources on real threats. But our approach goes beyond just technology. Here’s what sets us apart:
✅Continuous improvement
Our federated models continuously learn from diverse datasets across institutions. Unlike static rules-based systems, they adapt in real-time to evolving criminal strategies. By benefiting from collective intelligence, not just isolated experience, banks can stay ahead of sophisticated threats.
✅Integrated platform
Our secure platform orchestrates every step of the process. Each bank’s locally trained model contributes to a system where insights are aggregated and validated to create a more accurate “champion model.” This ensures best-in-class anomaly detection while preserving the privacy and security of sensitive data.
✅Behavioral intelligence
We combine cutting-edge AI with behavioral science to detect what other systems miss. By understanding how criminals operate, we help banks identify subtle, high-risk patterns that pure data analysis might overlook.
✅Future-ready
Financial crime keeps evolving, and so do we. From disintermediated payment channels to cross-border schemes and emerging technologies, our platform scales to meet tomorrow’s challenges. Banks gain a forward-looking solution that grows with them, helping them stay ahead of increasingly complex threats.
By combining advanced technology, human insight, and a scalable platform, Consilient offers banks a clear advantage in fighting financial crime. Rather than seeing us as “another tool”, banks refer to us as a partner.
Time to get ahead with better AML anomaly detection
Financial crime is growing more sophisticated every day, and traditional approaches just can’t keep up. But there’s a better way. Consilient’s Federated Learning platform helps banks share intelligence securely, reduce false positives, and catch more criminals.
Don’t just take our word for it. Our solution delivers 4x better detection rates and 75% improved efficiency at leading institutions—all while protecting sensitive data and meeting regulatory requirements.
Ready to strengthen your AML program? Let’s talk about how federated learning can help you catch more criminals while reducing costs. Contact us today.