From manual to machine learning: The evolution of AML compliance
We all know that the current approach to AML compliance is broken. Institutions that don’t prioritize AML innovation leave themselves vulnerable to losses. In fact, in 2023 one multinational bank was fined $186 million* by the US Federal Reserve.
But most organizations remain glued to their existing historic processes leaving them in the dark on the latest approaches. A more forward-thinking and progressive approach to AML system and controls, coupled with a solid understanding of the latest techniques will allow leaders to make smarter decisions about improving AML compliance.
In this post, we’ll explore the journey of AML compliance from its manual roots to the cutting-edge machine learning approaches of today. We’ll examine the strengths and limitations of each stage, and introduce federated learning—a technology that is redefining financial crime prevention.
Manual AML processes and rule-based systems
Despite advances in technology, firms still rely heavily on traditional rules-based systems, generating vast amounts of alerts, leading to an overwhelming number of false positives to pass to investigators for review.
Traditional rules-based transaction monitoring systems flag transactions based on fixed thresholds (for example, transactions over $10,000). This means rules-based systems often lack the flexibility to adapt to more complex and subtle money laundering patterns. As a result, they generate a large volume of false positives by flagging transactions that are legitimate but fall within predefined parameters.
Financial institutions process millions of transactions daily, making it difficult to differentiate between legitimate and suspicious activity in real time. Even with AI and machine learning, managing this massive volume without generating a large number of false positives is a challenge.
Analysts then meticulously review this high volume of records, manually.
This approach has been the norm for many years—and surprisingly, still persists in most financial institutions today. It’s painfully slow and prone to human error. In fact, U.S. financial institutions spend an estimated $180 million** annually on analyst salaries just for preparing Suspicious Activity Reports (SARs).
Limited customization means rule-based systems often apply the same criteria to all customers, without considering context such as customer behavior or industry-specific norms. This “one-size-fits-all” approach can trigger false positives for transactions that deviate slightly from a normal pattern but aren’t suspicious.
What’s more, as money launderers grow more sophisticated, they learn to structure their activities to slip through these static defenses.
Essentially, financial institutions find themselves in a constant game of cat and mouse, always one step behind.
While this approach had its limitations, it laid crucial groundwork. It highlighted the need for more intelligent, adaptable solutions in the world of financial crime prevention.
First-generation machine learning models
As the limitations of manual processes and rule-based systems became increasingly apparent, the industry turned to machine learning (ML). These local or in-house machine learning models have made significant advancements in AML compliance, boosting efficiency by 35%. Unlike their rigid predecessors, these models can analyze vast amounts of data, identify complex patterns, and adapt to new information.
However, it’s important to note that these early ML models weren’t without their own challenges. They often operated in data silos, limited to the information available within a single institution. This restricted their ability to identify broader patterns of financial crime that might span multiple organizations.
There’s also bias in training data. Machine learning models rely on historical data to learn what constitutes suspicious activity. If the training data used is biased or limited, the model may incorrectly label legitimate activities as suspicious, contributing to high false positives.
Plus, there’s the issue of overfitting. Sometimes, AI models may overfit to known patterns of money laundering, making them less effective at identifying novel or evolving techniques. This leads to low detection rates for new schemes.
What’s more, local ML models still generate a high number of alerts—around 600—many of which are likely false positives. This means that while the system is more efficient, AML teams are still dealing with a substantial volume of alerts to review.
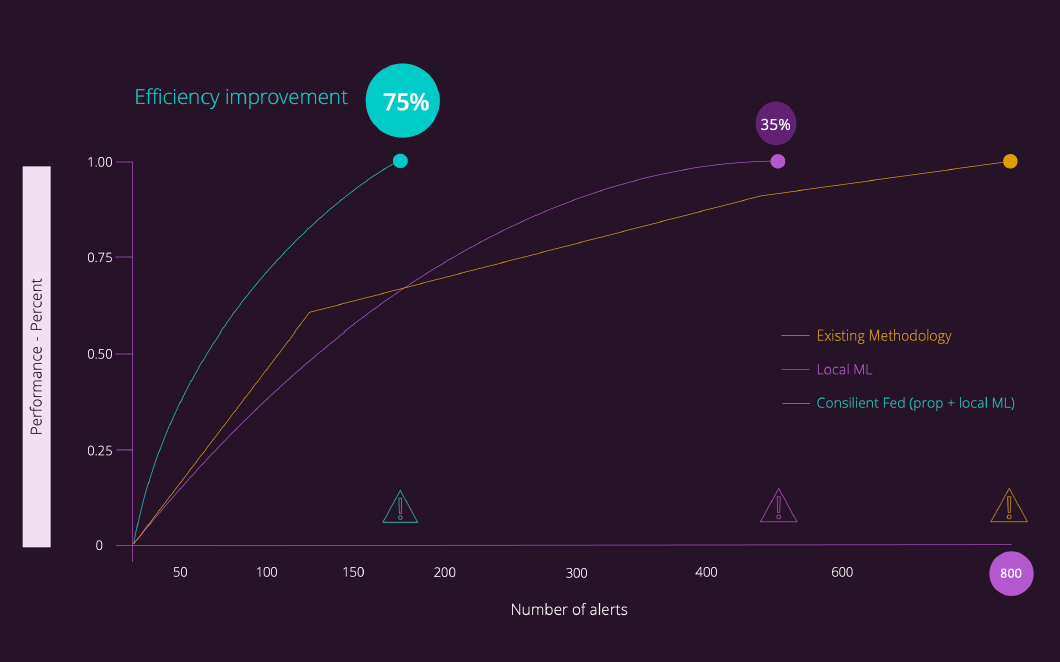
Another hurdle? Many financial institutions overestimated the capabilities of their ML models. They believed their systems were more accurate than they actually were, leading to a false sense of security.
Despite these limitations, the introduction of machine learning set the stage for even more advanced technologies that would push the boundaries of financial crime detection.
So, what came next in this technological evolution? Enter federated learning—a revolutionary approach that addresses many of the shortcomings of earlier ML models.
Federated learning approaches, like Consilient’s solution, show a dramatic improvement. As shown in the image above, not only do they increase efficiency by 75%, but they also significantly reduce the number of alerts to just under 200. This is a major leap forward in both efficiency and accuracy.
Federated learning: True innovation in AML compliance
As machine learning models became more sophisticated, a new challenge emerged: how to leverage collective knowledge without compromising data privacy. Despite advances in public-private partnerships, many financial institutions still work in isolation, limiting the overall effectiveness of AML efforts. Limited sharing of insights or best practices on emerging money laundering techniques can hinder effective detection.
This is where federated learning enters the picture.
Federated learning allows financial institutions to share behavioral insights—without sharing actual data. This collaborative approach enables the creation of more robust, accurate models for detecting financial crime.
Here’s a simple way to understand the difference:
- Centralized machine learning: Moves the data to the computation – DATA MOVES.
- Federated learning: Moves the computation to the data – MODEL MOVES.
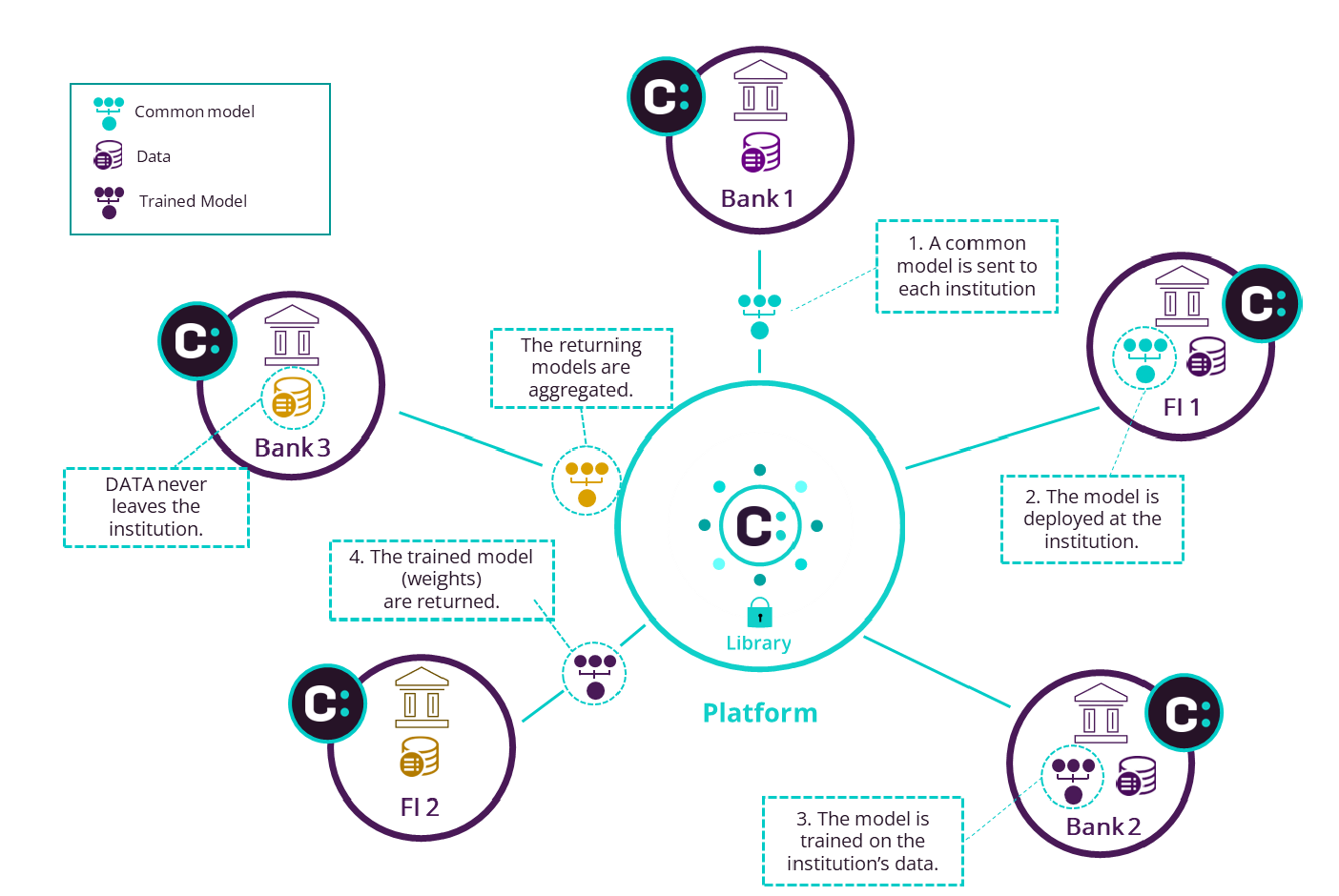
[Instead of moving data, Consilient’s federated learning algorithm travels from bank to bank]
The benefits are significant. Consilient clients find that our federated learning solution improves efficiency by 75%.
How does it work?
In essence, federated learning models are trained across multiple decentralized servers holding local data samples. Instead of exchanging data, only behavioral patterns derived from each set of data are shared. As the federated learning model travels, the data it learns from never leaves the owning organization.
This approach maintains data privacy while allowing institutions to benefit from a wider pool of information. This resulting model is said to be “federated” because it is trained on the combined learnings from all the models across different nodes.
For AML teams, this means access to market-wide learning and a diverse set of models. The result is improved identification of risks and suspicious activities, without the need to centralize sensitive financial data.
Federated learning also opens doors to standardized risk management across organizations. This is particularly valuable for large financial institutions with multiple divisions or those operating across different geographies.
Embracing the future of AML compliance with federated learning
As we’ve seen, federated learning offers a powerful solution to many longstanding issues in AML compliance. It combines the breadth of market-wide data with the privacy safeguards necessary in the financial industry. This technology promises more accurate detection of suspicious activities, improved efficiency, and standardized risk management across organizations.
By learning from multiple independent datasets, Federated Learning empowers banks to:
- Significantly reduce the number of false positive alerts,
- Lower operational costs,
- Improve the detection of criminal behavior using insights from a wide array of data rather than just their own company data,
- Identify new risks previously undetected by single organizations.
Financial institutions that adapt to these changes will be better equipped to detect and prevent financial crimes, reduce regulatory exposure, and operate much more efficiently.
As we move forward, one thing is clear: the evolution of AML compliance is not just about keeping pace with criminals—it’s about staying one step ahead.
Talk to us if you are keen to learn more about Federated Learning. We’d genuinely love to help.
Sources: https://gatenox.com/2023s-biggest-aml-fines/#:~:text=Deutsche%20Bank%20was%20fined%20%24186,the%20need%20for%20systemic%20improvements | https://www.bankdirector.com/article/the-high-cost-of-the-suspicious-activity-report/#:~:text=Suspicious%20activity%20reports%20(SARs)%20can,to%20do%20better%20for%20society.